Or try one of the following: 詹姆斯.com, adult swim, Afterdawn, Ajaxian, Andy Budd, Ask a Ninja, AtomEnabled.org, BBC News, BBC Arabic, BBC China, BBC Russia, Brent Simmons, Channel Frederator, CNN, Digg, Diggnation, Flickr, Google News, Google Video, Harvard Law, Hebrew Language, InfoWorld, iTunes, Japanese Language, Korean Language, mir.aculo.us, Movie Trailers, Newspond, Nick Bradbury, OK/Cancel, OS News, Phil Ringnalda, Photoshop Videocast, reddit, Romanian Language, Russian Language, Ryan Parman, Traditional Chinese Language, Technorati, Tim Bray, TUAW, TVgasm, UNEASYsilence, Web 2.0 Show, Windows Vista Blog, XKCD, Yahoo! News, You Tube, Zeldman
InfoWorld
Technology insight for the enterpriseWhat is serverless? Serverless computing explained 25 Dec 2024, 10:00 am
Over the past two decades, considerable innovation has gone into making sure developers don’t have to think much about the underlying physical machine their code runs on. Serverless computing is a paradigm that takes this objective to its logical conclusion. With serverless, you don’t have to know anything about the hardware or operating system your code runs on, as it’s all managed for you by a service provider. While the segment has had its ups and downs, it’s currently on the upswing: A recent report from SkyQuest technology projected that serverless computing would be a $50 billion market by 2031.
What is serverless computing?
Serverless computing is an execution model for the cloud in which a cloud provider dynamically allocates only the compute resources and storage needed to execute a particular piece of code. Naturally, there are still servers involved, but the provider manages the provisioning and maintenance. The user is charged for the resources consumed along with the maintenance and whatever other fees might be tacked on. In an ideal serverless scenario, there would be no servers to manage or provision at all: No bare metal, nothing virtual, no containers. Serverless users are not involved with managing a host, patching a host, or dealing with anything on an operating system level.
Serverless has been used to describe back-end-as-a-service scenarios, where a mobile app would connect to a back-end server hosted entirely in the cloud. But when people talk about serverless computing or serverless architectures, they usually mean function-as-a-service (FaaS), in which a customer writes code that only tackles business logic and uploads it to a provider. That’s our definition for this article: The provider handles all the hardware provisioning, virtual machine and container management, and even tasks like multithreading that often are built into application code. All that’s left to do is provide the functions that specifically implement the application’s business logic.
How serverless computing works
In a FaaS serverless environment, functions are event-driven, meaning the code is invoked only when triggered by a request. Requests can include things like API calls, HTTP requests, or file uploads; they could also be triggered by a scheduler if your application involves tasks that happen regularly.
You would deploy these functions on a specific serverless platform. As we’ll discuss below, there are many serverless providers to choose from, each with strengths and weaknesses. You would generally tailor the code for your function to the platform where you plan to deploy it. The provider charges only for compute time used by that execution, rather than a flat monthly fee for maintaining a physical or virtual server. These functions can be connected to create a processing pipeline, or they can serve as components of a larger application, interacting with other code running in containers or on conventional servers.
Benefits and drawbacks of serverless computing
Based on what you’ve read so far, the major benefits of serverless computing should be clear:
- Developers can focus on the business goals of the code they write, rather than on infrastructure questions. This simplifies and speeds up the development process and improves developer productivity.
- Organizations only pay for the compute resources they use in a very granular fashion, rather than buying physical hardware or renting cloud instances that mostly sit idle. That latter point is of particular benefit to event-driven applications that are idle much of the time but under certain conditions must handle many event requests at once. Rather than provisioning a beefy server that is underused most of the time, with a serverless architecture, you only pay for the server resources you need.
But there are a few drawbacks, as well:
- An obvious one is that serverless functions are intentionally ephemeral and often not suitable for long-term tasks. Most serverless providers won’t let your code execute for more than a few minutes, and when you spin up a function, it doesn’t retain any stateful data from previously run instances.
- A related problem is that serverless code can take as long as several seconds to spin up—not a problem for many use cases, but if your application requires low latency, be warned.
- Serverless functions also must be tailored to the specific platform they run on. This can result in vendor lock-in and less flexibility. Although there are open source options available, the serverless market is dominated by the big three commercial cloud providers (more about vendors shortly). Development teams often end up using tooling from their serverless vendor, which makes it hard to switch. And because so much of serverless computing takes place on the vendor’s infrastructure, it can be difficult to integrate serverless code into in-house development and testing pipelines.
Breaking down the pros and cons should provide insight into how serverless computing could be useful to you. It’s also helpful to be aware of the most common real-world applications for the technology:
- Batch processing of files or data. One of the canonical examples of a serverless architecture use case is a service that uploads and processes a series of individual image files and sends them along to another part of the application. These sorts of compute-intensive but only occasionally necessary bursts of activity are a great fit for the serverless pay-for-the-compute-you-use model.
- Scheduled or trigger-based tasks. Serverless functions can generate reports or backup images, send notifications, or perform other simple business logic, either in response to triggers delivered via APIs or HTTP calls or on a specified schedule.
- Automating CI/CD pipelines. Many of the tasks involved in automating the CI/CD pipelines that power devops are run from serverless platforms.
- Building RESTful APIs. Serverless platforms are a great foundation for RESTful API services because they can scale up cost effectively with use. The platforms provide infrastructure management along with high availability and reliability.
Serverless vendors: The big three and beyond
The modern age of serverless computing began with the launch of AWS Lambda, a platform based on Amazon’s cloud service. Microsoft followed suit with Azure Functions, and Google Cloud Functions reached production status after that. Martin Heller has a good rundown of serverless providers and how to choose a serverless platform. Offerings that have emerged in the past few years include Vercel Functions, Netlify Functions, Cloudflare Workers, and Fastify Serverless.
Also see: Serverless computing’s second act.
Serverless databases
One quirk of working with serverless code is that has no persistent state, which means the values of local variables don’t persist across instantiations. Any persistent data your code needs to access must be stored elsewhere. The triggers available in the stacks for the major vendors all include databases that your functions can interact with.
Some of these databases are themselves referred to as serverless. This means they behave much like other serverless functions discussed so far, with the obvious exception that data is stored indefinitely. But much of the management overhead involved in provisioning and maintaining a database is cast aside. As with function-as-a-service offerings, you only pay for the compute time you actually use, and resources are spun up and down as needed to match demand. Jozef de Vries discusses the pros and cons of a serverless database in Is a serverless database right for your workload?
The big three serverless providers each offer their own serverless databases: Amazon has Aurora Serverless and DynamoDB (the latter a NoSQL offering); Microsoft has Azure Cosmos DB, and Google has Cloud Firestore. These aren’t the only databases available, though, and much of what’s available is platform-agnostic. The list includes serverless variants of popular databases like CockroachDB and newer offerings catering to generative AI, such as the Pinecone Serverless vector database.
Serverless computing, Kubernetes, and Knative
Containers help power serverless technology under the hood, but the overhead of managing them is handled by the vendor and thus invisible to the user. Many see serverless computing as a way to get the advantages of containerized microservices without having to deal with their complexity. In fact, containers and serverless computing can complement each other, with serverless functions existing in the same application as containerized microservices.
Kubernetes, the most popular container orchestration platform, can enter the serverless world thanks to the open source extension Knative. With Knative, any container that runs on Kubernetes can be run as a serverless workflow in a way that’s transparent to container developers.
Knative is one example of a serverless framework—a set of infrastructure tools that provide the basis on which your code runs and does much of the work of interacting with the underlying platform. Unlike Knative, many serverless frameworks are tied to specific vendor platforms (there’s that vendor lock-in problem again) and will define much about how you end up building your application. Amazon has its own native offering, the open source Serverless Application Model (SAM), but there are others as well, some of which are cross-platform and also open source.
Get started with serverless computing
Starting out with serverless computing can be daunting, as it seems like you’d need to sign up with a vendor to even find out how it works. But fear not: There are ways to run serverless code offline on your own local hardware. For instance, the AWS SAM (Serverless Application Model) provides a Local feature that allows you to test AWS Lambda code offline. And, if you’re using the serverless application framework, check out serverless-offline, a plugin that lets you run code locally.
Happy experimenting!
Python in 2024: Faster, more powerful, and more popular than ever 25 Dec 2024, 10:00 am
Over the course of 2024, Python has proven again and again why it’s one of the most popular, useful, and promising programming languages out there. The latest version of the language pushes the envelope further for speed and power, sheds many of Python’s most decrepit elements, and broadens its appeal with developers worldwide. Here’s a look back at the year in Python.
Python 3.13 introduced the “no-GIL” build (and lots more)
In the biggest news of the year, the core Python development team took a major step toward overcoming one of Python’s longstanding drawbacks: the Global Interpreter Lock or “GIL,” a mechanism for managing interpreter state. The GIL prevents data corruption across threads in Python programs, but it comes at the cost of making threads nearly useless for CPU-bound work.
Over the years, various attempts to remove the GIL ended in tears, as they made single-threaded Python programs drastically slower. But the most recent no-GIL project goes a long way toward fixing that issue—enough that it’s been made available for regular users to try out.
The no-GIL or “free-threaded” builds are still considered experimental, so they shouldn’t be deployed in production yet. The Python team wants to alleviate as much of the single-threaded performance impact as possible, along with any other concerns, before giving the no-GIL builds the full green light. It’s also entirely possible these builds may never make it to full-blown production-ready status, but the early signs are encouraging.
Another forward-looking feature introduced in Python 3.13 is the experimental just-in-time compiler or JIT. It expands on previous efforts to speed up the interpreter by generating machine code for certain operations at runtime. Right now, the speedup doesn’t amount to much (maybe 5% for most programs), but future versions of Python will expand the JIT’s functionality where it yields real-world payoffs.
We kissed Python’s “dead batteries” goodbye
Python’s been around for over three decades, and it’s accumulated some cruft across the years. Some of that baggage consists of standard library modules that have fallen into obsolescence or are no longer actively maintained. Since Python is described as a “batteries-included” language, these aging modules have been called dead batteries.
Over the last couple of Python versions, numerous dead batteries have been deprecated and flagged for removal. With Python 3.13, they’re now entirely gone. It’s a step toward freeing Python from its legacy roots—but in some cases, removing those batteries broke other things that were still in use. The good news is, what’s dead and gone can and should be replaced—and it often isn’t hard to make the swap.
Python’s popularity topped the charts
Python’s enjoyed a massive uptake across the software development world in the last decade, but 2024 brought the biggest wins yet.
In 2024, Python climbed to 18% in the Tiobe index of programming languages, which rates how widely a language is used. The only other language to achieve that number was Java, back in 2016. It’s also the anticipated Tiobe programming language of the year. And finally, it’s now more widely used than JavaScript on GitHub. That last change can be attributed to Python’s central role in artificial intelligence, machine learning, and data science application code hosted on the platform.
Python is also recognized as one of the “big three” programming languages, as over the past three decades, enterprises have come to rely on Python, Java, and JavaScript above other languages. JavaScript is still the most supported way to do things in a web browser, and Java code runs a staggering amount of business logic and software infrastructure for enterprises. In addition to being the go-to platform for AI, machine learning, and data science (all crucial business functions now), Python’s big strength is its versatility. It’s effectively the second-best language for just about anything, and also the most convenient and quickest-to-use language for nearly everything. That dream has been coming true for a long time now, and it stands to be true for a long time to come.
The AI backlash couldn’t have come at a better time 24 Dec 2024, 10:00 am
At a developers conference I attended not too long ago, attendees did little to hide their disdain every time the term “AI” was bandied about. (And it was bandied about a lot!) So I was careful on a recent call attended by about 250 engineers to preface the AI portion of the discussion with, “I know this will make you cringe, but…” That got a lot of knowing laughs and thumbs-up emojis.
What’s going on here? It’s not that these developers and engineers are against the use of AI; it’s that they are tired of hearing about artificial intelligence as a panacea without pragmatism. They want to hear about how they can pragmatically and easily harness it — now — for real-life use cases.
Indeed, we’ve spent the last few years bombarded by hyperbolic talk about AI (Robotaxis anyone?). How it’s going to transform life as we know it. How it’s going to take our jobs. When it will become sentient…
Meanwhile, AI has kind of quietly become part of the fabric of our lives — not by changing our lives or taking our jobs or becoming sentient, but by making our lives and our jobs easier. For example, when I Googled “When will AI become sentient?” (and “When did Skynet become self-aware,” for comparison purposes), I didn’t have to comb through results one at a time but instead read the AI-generated summary of the most relevant content at the top, with sources. (Spoiler alert: Opinions are mixed.)
There are hundreds of other examples of AI applications that are, well, pretty boring but really useful. What’s a lot less boring right now is scaling and integrating AI across the organization. And that’s where the AI backlash can be leveraged.
Making AI usefully boring
Developers, engineers, operations personnel, enterprise architects, IT managers, and others need AI to be as boring for them as it has become for consumers. They need it not to be a “thing,” but rather something that is managed and integrated seamlessly into — and supported by — the infrastructure stack and the tools they use to do their jobs. They don’t want to endlessly hear about AI; they just want AI to seamlessly work for them so it just works for customers.
Organizations can support that by using tools that are open, transparent, easy to use, compatible with existing systems, and scalable. In other words, boring.
The open source RamaLama project’s stated goal, for example, is to make AI boring through the use of OCI containers. RamaLama facilitates the ability to locally discover, test, learn about, and serve generative AI models — in containers. It first inspects your system for GPU support, defaulting to CPU support if no GPUs are present. It then uses either Podman or Docker (or runs on the local system if neither are present) to pull the OCI image you want with all the software needed to run an AI model with your system’s setup. This eliminates the need for users to perform complex configurations on their systems for AI.
The Ollama project similarly helps users get up and running with AI models locally, but it doesn’t help you run in production. RamaLama goes a step further by helping you push that model into a container image and then push that container image out to a registry. Once you have a container image, you can ship it off, fine-tune it, and bring it back. It gives you the portability of containers for model development.
(My colleagues Dan Walsh and Eric Curtin posted a great video on YouTube that puts RamaLama in perspective, along with a demo.)
RamaLama isn’t the only project or product that can support AI humdrumness, but it’s a great example of the kinds of things to look for when adopting AI systems across the organization.
Right-sizing the models
The models themselves are also, rightly, growing more mainstream. A year ago they were anything but, with talk of potentially gazillions of parameters and fears about the legal, privacy, financial, and even environmental challenges such a data abyss would create.
Those LLLMs (literally large language models) are still out there, and still growing, but many organizations are looking for their models to be far less extreme. They don’t need (or want) a model that includes everything anyone ever learned about anything; rather, they need models that are fine-tuned with data that is relevant to the business, that don’t necessarily require state-of-the-art GPUs, and that promote transparency and trust. As Matt Hicks, CEO of Red Hat, put it, “Small models unlock adoption.”
Similarly, organizations are looking for ways to move AI from the rarefied air of data science to a place where stakeholders across the organization can understand and make use of it as part of their day-to-day work. For developers, this kind of democratization requires tools that enable safe spaces for experimentation with building, testing, and running intelligent applications.
Here’s a provocative premise: LLMs and models are just software. They’re just files and processes, processes which run on CPUs and GPUs.
It just so happens that we have a technology that can help with files and processes — Linux containers. Linux is the default platform for AI development, so it makes sense to use Linux containers, which give developers a safe place to experiment without necessarily putting their data in the cloud. Containers also give developers an easy way to move their applications from those safe spaces to production, without having to worry about infrastructure.
A home in containers
Organizations already have container infrastructure like registry servers, CI/CD testing, and production tools like Kubernetes, with key capabilities like scalability, security, and Linux optimizations for running predictive and generative AI workloads. This approach empowers teams to effectively leverage AI capabilities while maintaining flexibility and control over their data across diverse environments.
The AI wave is no different than that surrounding other transformative technologies. (And, yes, I said it, it is transformative.) Think about how we used to say web-based or cloud-based before everything just became web-based or cloud-based and we didn’t need to add the modifier anymore. But that happened only after similar backlash and efforts to make web- and cloud-based technology more usable. The same will (and is) happening with AI.
—
Generative AI Insights provides a venue for technology leaders—including vendors and other outside contributors—to explore and discuss the challenges and opportunities of generative artificial intelligence. The selection is wide-ranging, from technology deep dives to case studies to expert opinion, but also subjective, based on our judgment of which topics and treatments will best serve InfoWorld’s technically sophisticated audience. InfoWorld does not accept marketing collateral for publication and reserves the right to edit all contributed content. Contact doug_dineley@foundryco.com.
Overcoming modern observability challenges 23 Dec 2024, 10:00 am
Observability, at its core, is about collecting and analyzing data generated from applications, cloud computing resources, and edge devices to ensure software and services are run smoothly, user experiences are optimized, and resources are managed cost-effectively. According to a recent Gartner report, 70% of companies that successfully apply observability will gain a competitive edge through faster problem identification and enhanced decision-making.
However, as application development has progressed from monolith stacks to microservices, and user expectations have shifted toward instant digital experiences, companies have accumulated a mix of observability tools, each tailored to different parts of their tech stack. This hodgepodge raises costs, complicates data analysis, and slows issue resolution.
The Kloudfuse observability platform addresses these challenges by building on three foundational principles: unification, cost optimization, and intelligent automation. Here’s a closer look at the observability landscape, the unique challenges organizations face today, and how Kloudfuse is designed to provide a unified, affordable, and intelligent approach to modern observability.
Observability challenge #1: Fragmentation and complexity
Traditionally, organizations have deployed multiple observability tools across their technology stacks to address distinct needs like monitoring logs, metrics, or traces. While these specialized tools excel individually, they rarely communicate well, resulting in data silos. This fragmentation prevents teams from gaining comprehensive insights, forcing devops and SRE (site reliability engineering) teams to rely on manual integrations to piece together a full picture of system health. The outcome is delayed insights and an extended mean time to resolution (MTTR), slowing down effective issue response.
Additionally, organizations now need to incorporate data streams beyond the traditional MELT (metrics, events, logs, and traces) framework, such as digital experience monitoring (DEM) and continuous profiling, to achieve comprehensive observability. DEM and its subset, real user monitoring (RUM), offer valuable insights into user interactions, while continuous profiling pinpoints low-performing code. Without integrating these data streams, teams struggle to link customers’ real experiences with specific code-level issues, resulting in data gaps, delayed issue detection, and dissatisfied customers.
Observability challenge #2: Escalating costs
The cost of observability has surged alongside the fragmentation of tools and the growing volume of data. SaaS-based observability solutions, which manage data ingestion, storage, and analysis for their customers, have become particularly expensive, with costs quickly accumulating. According to a recent IDC report, nearly 40% of large enterprises view high ownership costs as a major concern with observability tools, with the median annual spend by large organizations (10,000+ employees) on AIops and observability tools reaching $1.4 million.
Additionally, data transfer fees—commonly known as egress fees—have been a significant concern, as companies must pay for data leaving their platforms and entering SaaS observability clouds. When combined with pay-per-use pricing models, this often leads to unexpected budget overruns for enterprises. It’s no surprise that businesses are becoming increasingly frustrated with high SaaS observability costs, vendor lock-in, and the lack of deployment flexibility.
Observability challenge #3: Intelligence and insight
Observability is crucial for quickly detecting issues and taking corrective actions to ensure that application performance does not negatively impact customer experience. With millions of transactions occurring every second, relying on traditional logic, predefined rules, and human intervention is no longer sufficient. According to a 2023 Gartner report, applied observability has emerged as one of the top 10 strategic technology trends, underscoring the increasing need for using AI to make smarter, more automated solutions to stay competitive and optimize business operations in real time.
Today’s observability solutions must go beyond static monitoring by incorporating AI and machine learning to detect patterns, trends, and anomalies. By automatically identifying outliers and emerging issues, AI-driven systems reduce the mean time to detect (MTTD) and mean time to resolve (MTTR), driving efficiency and helping teams address potential problems before they affect end-users.
A unified, cost-effective, and intelligent platform
Recognizing these challenges, Kloudfuse set out to build an observability platform that addresses the specific needs of modern cloud-native applications and dynamic digital experiences.
Unified observability
Kloudfuse brings together diverse data streams in a single platform, eliminating the need for multiple observability tools and manual data stitching. By unifying metrics, events, logs, and traces in its observability data lake, Kloudfuse empowers teams with a full-stack, unified approach to observability, enabling seamless correlation of front-end and back-end issues to significantly reduce MTTR.
With Kloudfuse 3.0, the observability data lake extends to integrate traditional MELT data with digital experience monitoring and continuous profiling, giving enterprises a comprehensive view of both user experience and system performance.
Real user monitoring delivers in-depth visibility into user interactions across every digital transaction—from front end to back end—enhanced with pixel-perfect replays of user journeys, providing full context for troubleshooting. Continuous profiling enables granular, line-level breakdowns of resource hot spots that cause application delays. This helps developers quickly debug and resolve performance bottlenecks for more efficient and reliable code. Additionally, continuous profiling identifies resource issues, such as CPU utilization and memory allocation, helping to reduce costs—a pillar of Kloudfuse discussed in the following section.
Cost optimization
Kloudfuse is designed with affordability and flexibility at its core. Unlike SaaS solutions that charge for data overages, Kloudfuse offers versatile deployment options, including private deployment in Amazon Web Services, Microsoft Azure, and Google Cloud Platform environments. This versatility not only avoids the high fees typical of SaaS and eliminates egress and data transfer fees, but also grants greater control over data residency—essential for organizations with strict data sovereignty requirements. Kloudfuse 3.0 expands the platform’s VPC private deployment model to support Arm processors, ensuring even further cost reduction and efficiency required by large-scale observability deployments.
The Kloudfuse platform offers additional cost-saving features, such as patent-pending fingerprinting technology that automatically detects patterns in log messages to reduce storage and processing requirements, driving down associated costs. In Kloudfuse 3.0, the log archival and hydration feature allows customers to store logs more affordably using compressed JSON formats within the customer’s own storage, such as Amazon S3, meeting compliance standards without excessive expenses.
Additionally, Kloudfuse 3.0 includes cardinality analysis, metric roll-ups, and data shaping and transformation capabilities that convert high-volume data into valuable attributes, further reducing both storage and analysis costs.
Intelligent automation
Kloudfuse’s integrated AI and machine learning capabilities enable teams to detect anomalies, trends, and root causes quickly. Advanced algorithms like rolling quantile, SARIMA, DBSCAN, seasonal decomposition, and Pearson correlation provide smarter alerting by pinpointing outliers, forecasting potential issues, and correlating observability data across streams to more quickly identify dependencies and root causes. The platform’s analytics tools also allow teams to visualize service dependencies and relationships with interactive service maps and topology views.
Kloudfuse 3.0 introduces Prophet for anomaly detection and forecasting to provide more accurate results, managing irregular time series that include missing values, such as gaps from outages or low activity, resulting in less tuning and improved forecasting, even with limited training data.
Kloudfuse 3.0 also introduces features like K-Lens and FuseQL to further enhance incident response and investigation. K-Lens uses outlier detection to quickly analyze thousands of attributes within high-dimensional data, identifying those that cause specific issues. It then uses heat maps and multi-attribute charts to pinpoint the sources of these issues, accelerating debugging and incident resolution. FuseQL offers a more expressive query language than LogQL, enabling advanced log analysis with multi-dimensional aggregations, filters, and anomaly detection, and supporting complex alerting conditions.
The future of observability
Organizations need an observability solution that is comprehensive, cost-effective, and intelligent. The Kloudfuse observability platform is designed to monitor modern cloud-native workloads while optimizing costs, offering insights into model performance and mitigating risks. Kloudfuse also leverages AI and machine learning to enhance data analysis, improving alerting, issue resolution, and performance optimization. Recognizing the need for AIops and LLMops, Kloudfuse is set to enable proactive monitoring of generative AI applications, ensuring reliability and business continuity.
As observability becomes a strategic asset, Kloudfuse provides a unified, scalable solution, expanding to support emerging use cases and harnessing AI to continually improve platform intelligence.
Pankaj Thakkar is co-founder and CTO of Kloudfuse.
—
New Tech Forum provides a venue for technology leaders—including vendors and other outside contributors—to explore and discuss emerging enterprise technology in unprecedented depth and breadth. The selection is subjective, based on our pick of the technologies we believe to be important and of greatest interest to InfoWorld readers. InfoWorld does not accept marketing collateral for publication and reserves the right to edit all contributed content. Send all inquiries to doug_dineley@foundryco.com.
How generative AI could aid Kubernetes operations 23 Dec 2024, 10:00 am
Enterprises often encounter friction using Kubernetes (K8s for short) at scale to orchestrate large swaths of containers, not to mention an escalating number of clusters. And due to its complexity, diagnosing issues within Kubernetes isn’t all that easy. At the same time, IT is looking at AI to automate aspects of configuring, managing, and debugging complex back-end technologies.
“Trying to solve IT problems with AI is nothing new,” says Itiel Schwartz, co-founder and CTO at Komodor. “It typically overpromises and underdelivers.” Yet, although he was skeptical at first, he now sees promise in utilizing finely-tuned generative AI models to reduce barriers and streamline Kubernetes operations.
Fine-tuned AI for root cause analysis
Accuracy in AI models hinges on their training data sets. And today’s popular large language models (LLMs), like OpenAI’s GPT, Meta’s Llama, Anthropic’s Claude, or Google’s Gemini, are trained on vast corpora of text data. While this works for general-purpose use, they often produce irrelevant recommendations for ultra-specific devops functions, says Schwartz.
Instead of using catch-all models, Schwartz believes narrow models are better for diagnosing Kubernetes issues. They can help avoid AI hallucinations or errors by following a more authoritative, controlled process—like fetching one piece of highly relevant data, like logs, metrics, or related changes.
One such tool is Komodor’s KlaudiaAI, an AI agent narrowly trained on historical investigations into Kubernetes operational issues. KlaudiaAI is trained for root cause analysis and excels at identifying an issue, sourcing relevant logs, and offering specific remediation steps. For example, when an engineer encounters a crashed pod, KlaudiaAI might correlate this to an API rate limit found in the logs and suggest setting a new rate limit.
Using AI to automate K8s management
Of course, Komodor isn’t the only company investigating the use of AI agents and automation to streamline Kubernetes management. K8sGPT, an open-source Cloud Native Computing Foundation (CNCF) sandbox project, uses Kubernetes-specific analyzers to diagnose cluster issues and respond with remediation advice in plain English. Robusta is a similar AI copilot designed for Kubernetes troubleshooting, such as incident resolution and alerts. Cast AI uses generative AI to auto-scale Kubernetes infrastructure to reduce operating expenses.
And if we look at the major cloud service providers, ChatOps is nothing new. For instance, Amazon offers AWS Chatbot, which can provide alerts and diagnostic information on Amazon Elastic Kubernetes Service workloads and configure resources based on chat commands. Amazon also has Amazon Q, an AI assistant with a variety of skills including building on the AWS cloud, through it’s not specifically geared toward K8s management.
Similarly, Google’s generative AI assistant, Gemini, serves as an all-purpose tool for Google Cloud, and is not specifically bred for remediating Kubernetes issues. However, Google Kubernetes Engine is optimized for training and running AI/ML workloads, and its GKE Autopilot can optimize the performance of infrastructure. A Kubernetes-focused AI assistant may not be far behind.
Other major cloud players are also looking to cash in on generative AI, notably in the monitoring and observability space. Last year, Datadog introduced Bits AI, a devops copilot designed for incident response across any data source Datadog touches. Bits AI can go deep to surface traces and logs and provide remediation advice for incident resolution.
Yet, the ongoing problem, Schwartz says, is that most of the AI models in the enterprise IT market still cast too wide a net with their training sets to be useful for the specific area of Kubernetes diagnosis. “If you use a generic AI model to investigate these issues, it will simply fail you. We tried it time after time,” Schwartz says. “As you narrow the scope down, the possibility of hallucinations goes down.”
That said, a heightened attention to detail can bring downsides. For example, Schwartz notes that Klaudia is often slower than other models (it may take 20 seconds to come up with an answer). This is because it privileges accuracy over speed, using an iterative investigation process until the root cause is completed. The good news is that by incorporating more sanity checks, the model improves accuracy, he says.
Reducing barriers to K8s usability
Kubernetes is the undisputed infrastructure layer for modern IT. Impressively, 84% of respondents to CNCF’s 2023 annual survey said they were using or evaluating Kubernetes. And, much headway has been made in optimizing Kubernetes for AI/ML workloads. “One of the biggest reasons for migrating to K8s is the ability to run more efficient ML,” says Schwartz.
Yet, security, complexity, and monitoring rank as the topmost challenges in using or deploying containers for heavily cloud-native organizations. According to PerfectScale, common issues, such as not setting memory limits, not properly allocating RAM for pods, or not setting CPU requests, threaten the reliability of Kubernetes. Now, the question is if generative AI can help operators, like platform engineers or site reliability engineers, better interface with the platform.
“Gen AI is not really production grade in most companies,” says Schwartz, who acknowledges its limitations and that it tends to work best in human-in-the-loop scenarios. Nevertheless, he foresees AIOps soon to become a helpful ally for addressing root cause, misconfigurations, and network issues and for guiding optimizations.
Kubernetes-specific, finely-tuned AIs could help operators more quickly diagnose problems, like failed deploys or failed jobs, and tie them to root causes when they arise. “Gen AI is going to take this toil and automate it,” Schwartz says.
The exciting new world of Redis 23 Dec 2024, 10:00 am
If you’re a developer who wants the most feature-rich, high-performance version of Redis, your choice is clear: Redis and not a fork. If you have the time and inclination to dabble in ideological debates about open source licensing, well, you might make another choice. But if you’re just trying to get your job done and want a great database that historically was primarily a cache but today offers much more, you’re going to opt for Redis and not its fork, Valkey.
So argues Redis CEO Rowan Trollope in an interview. “It is unquestionable that Redis, since we launched Redis 8.0 with all the capabilities from Redis Stack, is just a far more capable platform,” he says. He substantiates the claim by cataloging “a whole bunch of things” that Valkey doesn’t offer, at least not at parity: vector search, a real-time indexing and query engine, probabilistic data types, JSON support, etc. (Note that some vendors, like Google Cloud, have started to fill in some of these blanks, at least in pre-GA releases, like Google’s Memorystore.)
That’s all CEO-speak, right? What would a serious technologist say about Redis? It might be difficult to find a more credible Redis expert than Redis founder Salvatore Sanfilippo who recently returned to the Redis community (and company) he left in 2020. Why return? Among other reasons, Sanfilippo wants to help shape Redis for a world awash with generative AI. In his words, “Recently I started to think that sorted sets can inspire a new data type, where the score is actually a vector.” Trollope says, “Redis has a real opportunity to emerge as a core part of the genAI infrastructure stack.” Discussions about licensing, Trollope notes, might be fun “popcorn fodder” that fixates on the past, but the real focus should be on Redis’ future as an integral part of the AI stack.
Forking for the wrong reasons
We live in a weird time when a few trillion-dollar companies get away with pleading poverty, arguing that they should be gifted a wealth of open source software without helping sustain its success. “Somehow Amazon and Google positioned themselves as the open source–friendly companies, which seems to be the opposite of reality,” Trollope tells me. In response, companies like Redis have gone through all sorts of licensing and packaging gymnastics (e.g., Redis Stack) to try to remain open to everyone except the clouds that threatened their ability to continue building and releasing open source software.
Did Redis manage this process perfectly? No. As Trollope tells me, “We didn’t effectively communicate with the community about our motivations and what we were doing and why we were doing it.” He continues, “I’m sure we can do things differently and better, and that’s our goal going forward.” For his part, Sanfilippo writes, “I don’t believe that openness and licensing are only what the OSI [Open Source Initiative] tells us they are,” but rather “a spectrum of things you can and can’t do.” It’s a nuanced view on a nuanced topic that too often gets painted in black and white.
Though portrayed as motivated by ideological differences over open source versus closed source software, ultimately AWS and Google forked Redis to further their business needs. Named Valkey, this Redis fork isn’t Redis, however much Valkey’s commercial backers cling to the “Redis compatible” tagline, and it will become more distinct over time. That’s a good thing. After all, Valkey has developers like Madelyn Olson who can turn it into something amazing. Recently, for example, AWS made significant improvements to Valkey’s scalability and memory efficiency. This will play out like AWS’s fork of Elasticsearch, OpenSearch, which is becoming a compelling product in its own right and not just a pale shade of Elasticsearch. It’s a great thing when the clouds create, rather than copy, open source.
Ultimately, very few developers care about open source ideological debates. As Trollope suggests, “If you’re the average developer, what you really care about is capability: Does this thing offer something unique and differentiated … that I need in my application?” On that note, he continues, “Pound for pound, we’ve been able to drive innovation dramatically more quickly than Valkey has.” Even if you disagree with that assessment, it’s hard to overlook just how differently Redis and the Valkey community see their futures, which brings us back to Sanfilippo and AI.
A different future for Redis
The Valkey development community isn’t focused on building and positioning it for generative AI workloads. Redis, by contrast, definitely hopes to ensure Redis’s place in the modern AI-centric stack. In such a world, debates about Redis licensing are “interesting but not that helpful,” Trollope argues. What really matters is whether developers can easily use Redis to build something awesome.
Sanfilippo, for his part, isn’t new to AI. “I wrote my first [neural network] library in 2003 and was totally shocked by how powerful and cool the whole concept was,” he writes. But “now, at the end of 2024, I’m finally seeing incredible results in the field. Things that looked like sci-fi a few years ago are now possible.” Although impressed by large language models such as Claude, Sanfilippo is actively working on novel approaches to Redis, like vector sets, which is “exactly the idea of sorted sets, but with multi-dimensional scores (embeddings!) and K-NN [k-nearest neighbor] matches.”
It’s a cool way to bring a very Redis flavor to generative AI. But among Sanfilippo’s interest in exploring “new ideas that can be exciting,” it’s hard to think of anything more exciting than Redis’s founder coming back to help shepherd its future. Imagine you’re an outsider without any knowledge of the past few years of licensing debates and you’re placing bets on the Redis-esque product most likely to win over developers. Against that backdrop, if “you take a guy like Salvatore now being a key contributor and leader of Redis, look, I’d make that bet every day of the week,” argues Trollope. Many developers likely will too.
Cost-conscious repatriation strategies 20 Dec 2024, 10:00 am
In recent years, the cloud computing landscape has undergone a seismic shift. After fully embracing the potential of public cloud services, many companies are reevaluating their strategies and shifting workloads back to on-premises infrastructures.
This trend, known as cloud repatriation, is driven by rising costs and increasing complexities in public cloud environments. More and more repatriation projects are underway as enterprises continue to receive exorbitant cloud bills. Many enterprises now realize that the unexpectedly high costs of cloud services are unsustainable in the long term.
GEICO has made headlines for its decision to repatriate numerous workloads back to on-premises solutions. In a recent interview, Rebecca Weekly, the vice president of platform and infrastructure engineering, said the initial goal of moving to the cloud was to reduce costs and complexity, but it ultimately had the opposite effect.
After a decade of cloud investments, GEICO’s bills spiked at 2.5 times the anticipated costs and the company faced increased reliability challenges. This tracks with what I’ve written in my book, An Insider’s Guide to Cloud Computing. These are the typical problems enterprises face when they use a haphazard lift-and-shift migration strategy that fails to align legacy systems with cloud efficiencies.
GEICO is not an isolated case. Across industries, organizations are discovering that data storage in the public cloud can be prohibitively expensive, mainly when their operational needs rely on vast amounts of data that require efficient and affordable management.
For enterprises operating in sectors such as finance or insurance, regulatory compliance further complicates matters. Companies must retain enormous volumes of sensitive data for long periods, often resulting in sky-high cloud storage expenses. Approaches to cloud computing that were once considered revolutionary are now viewed as a potential liability.
Rethinking cloud
First, this is not a pushback on cloud technology as a concept; cloud works and has worked for the past 15 years. This repatriation trend highlights concerns about the unexpectedly high costs of cloud services, especially when enterprises feel they were promised lowered IT expenses during the earlier “cloud-only” revolutions.
Leaders must adopt a more strategic perspective on their cloud architecture. It’s no longer just about lifting and shifting workloads into the cloud; it’s about effectively tailoring applications to leverage cloud-native capabilities—a lesson GEICO learned too late. A holistic approach to data management and technology strategies that aligns with an organization’s unique needs is the path to success and lower bills.
Organizations are now exploring hybrid environments that blend public cloud capabilities with private infrastructure. A dual approach, which is nothing new, allows for greater data control, reduced storage and processing costs, and improved service reliability. Weekly noted that there are ways to manage capital expenditures in an operational expense model through on-premises solutions. On-prem systems tend to be more predictable and cost-effective over time.
Moreover, the rise of open source technologies provides a pathway for companies seeking to modernize their infrastructure. The open source approach avoids burdensome costs associated with traditional public cloud providers. By leveraging frameworks like Kubernetes for container orchestration and OpenStack for private cloud deployments, businesses can regain control over their data and resources while minimizing dependence on public cloud offerings. Of course, this comes with its own set of cautions. Containers cost more than traditional development. Still, when deployed on owned hardware, you’ve already paid for the excess memory, processor, and storage resources they use.
A trend or just a few cases?
The current rash of cloud repatriations is emblematic of a broader reevaluation of how businesses approach their technological infrastructure. The era of indiscriminate cloud adoption is giving way to a more tempered and pragmatic approach. We’ve learned that it pays to incorporate operational costs, compliance requirements, and specific business use cases into infrastructure design.
Many cloud providers don’t know they have a repatriation problem because it has not yet impacted revenue. They’re also distracted by the amount of AI experimentation going on, which has a massive impact on public cloud providers’ services. However, if cloud providers don’t get a clue about the assistance their clients need to reduce cloud costs, we’ll see more and more repatriation in the next several years.
Technology leaders must now, more than ever, comprehensively understand their company’s unique data landscape and leverage cost-effective solutions that empower their business. That seems like common sense, but I’m disturbed by the number of firms that blindly adopt cloud computing without adequately understanding its impact. Many are now hitting a cloud wall for reasons that have nothing to do with cloud technology’s limitations; they’re the results of bad business decisions, plain and simple. Expect more companies to follow GEICO’s lead as we advance into 2025.
Smart policing revolution: How Kazakhstan is setting a global benchmark 20 Dec 2024, 5:08 am
In the era of digital transformation, public safety stands at a critical crossroads. Law enforcement agencies globally are under increasing scrutiny to enhance transparency, efficiency, and trust within their communities. Against this backdrop, Kazakhstan’s “Digital Policeman” initiative has emerged as a shining example of technological innovation in policing.
The initiative leverages state-of-the-art technologies like smart badges and military-grade mobile devices, designed to empower officers while ensuring accountability. These smart badges go beyond conventional body cameras, offering features such as continuous, tamper-proof video recording, GPS tracking, encrypted data handling, and emergency alert systems. This cutting-edge approach has turned routine policing into a sophisticated operation backed by real-time data and insights.
Why it matters: Key impacts
The numbers speak volumes. Since its inception, the Digital Policeman project has documented over 6,000 bribery attempts, recorded 443,765 administrative violations, and solved 2,613 crimes—all while saving Kazakhstan’s national budget $6 million. With over 10,000 smart badges and 21,000 tablets deployed, the project is reshaping the very fabric of public safety.
These advancements extend beyond technology. By addressing the limitations of traditional tools, such as unreliable video recorders prone to tampering, the project has reduced corruption, streamlined workflows, and enhanced officer safety. Moreover, officers now have access to tools that enable quicker decision-making and more effective resource allocation, fostering greater community trust.
Global leadership in law enforcement innovation
The success of the Digital Policeman initiative positions Kazakhstan as a leader in police modernization, standing shoulder-to-shoulder with global pioneers like the United States, Korea, and Scotland. The initiative’s integration of secure, military-grade technology sets a benchmark, inspiring other nations, including Azerbaijan, Kyrgyzstan, and Uzbekistan, to explore similar advancements.
Looking ahead: The future of public safety
Kazakhstan is not stopping here. The initiative is poised for expansion, with plans to incorporate advanced features like facial recognition and direct integration with law enforcement databases. These enhancements will further amplify operational efficiency, enabling real-time communication of alerts and seamless access to critical information during patrols.
Beyond policing, this technology holds potential applications in other public safety domains such as traffic management, fisheries supervision, and forestry oversight. By extending the reach of smart badges, Kazakhstan continues to redefine the possibilities of public safety in the digital age.
Why read the full spotlight paper?
This article only scratches the surface of the profound impact the Digital Policeman project is making. For a comprehensive look at the strategies, technologies, and lessons learned from this groundbreaking initiative, download the full spotlight paper. Discover how Kazakhstan is charting the future of law enforcement, balancing technological innovation with community trust.
Unlock the future of policing. Download the full report today!
Go 1.24 brings full support for generic type aliases 19 Dec 2024, 10:00 am
Go 1.24, a planned update to Google‘s popular open-source programming language, has reached the release candidate stage. Expected to arrive in February 2025, the release brings full support for generic type aliases, along with the ability to track executable dependencies using tool
directives in Go modules.
Draft release notes for Go 1.24 note that the release brings full support for generic type aliases, in which a type alias may be parameterized like a defined type. Previously, A type alias could refer to a generic type, but the type alias could not have its own parameters. For now, generic type aliases can be disabled by setting GOEXPERIMENT=noaliastypeparams
. This parameter setting will be removed in Go 1.25.
With the go
command in Go 1.24, Go modules now can track executable dependencies using tool
directives in go.mod files. This removes the need for a previous workaround of adding tools as blank imports to a file conventionally named tools.go
. The go tool
command now can run these tools in addition to tools shipped with the Go distribution. Also with the go
command, a new GOAUTH
environment variable offers a flexible way to authenticate private module fetches.
Cgo, for creating Go packages that call C code, now supports new annotations for C functions to improve runtime performance. With these improvements, #cgo noescape cFunctionName
tells the compiler that memory passed to the C function cFunctionName
does not escape. Also, #cgo nocallback cFunctionName
tells the compiler that the C function cFunctionName
does not call back to any Go functions.
Other new features and improvements in Go 1.24:
- Multiple performance improvements to the runtime in Go 1.24 have decreased CPU overheads by 2% to 3% on average across a suite of representative benchmarks.
- A new
tests
analyzer reports common mistakes in declarations of tests, fuzzers, benchmarks, and examples in test packages, such as incorrect signatures, or examples that document non-existent identifiers. Some of these mistakes might cause tests not to run. - The
cmd/go
internal binary and test caching mechanism now can be implemented by child processes implementing a JSON protocol between thecmd/go
tool and the child process named by theGOCACHEPROG
environment variable. - An experimental
testing/synctest
package supports testing concurrent code. - The debug/elf package adds several new constants, types, and methods to add support for handling dynamic versions and version flags in ELF (Executable and Linkable Format) files.
- For Linux, Go 1.24 requires Linux kernel version 3.2 or later.
Go 1.24 follows Go 1.23, released in August, featuring reduced build times for profile-guided optimization.
Create searchable Bluesky bookmarks with R 19 Dec 2024, 10:00 am
The Bluesky social network is seeping into the mainstream, thanks in part to people unhappy with changes at X (formerly Twitter). “It’s not some untouched paradise, but it’s got a spark of something refreshing,” Jan Beger, global head of AI Advocacy at GE HealthCare, posted on LinkedIn last month. “Like moving to a new neighborhood where the air feels a little clearer, and people actually wave when they see you.”
That includes an increasing number of R users, who often use the hashtag #rstats. “Bluesky is taking off! Please join us there with this handy Posit employees starter pack,” Posit (formerly RStudio) wrote in its December newsletter. Starter packs make it easy to find and follow other Bluesky users based on topics and interests, either one by one or with a single click. Any user can create them. (See my companion FAQ on Bluesky with tips for getting started.)
However, because Bluesky is relatively new (it opened to the general public early this year), some features that are standard on other microblogging platforms aren’t yet available. One of the most sorely missed is “bookmarks” — being able to save a liked post for easy retrieval later. There are a couple of workarounds, either with third-party apps or custom feeds. In addition, thanks to Bluesky’s free API and the atrrr R package, you can use R to save data locally of all your liked posts — and query them however you want. Here’s how.
Save and search your Bluesky likes
This project lets you download your Bluesky likes, wrangle the data, save it, and load it into a searchable table. Optionally, you can save the data to a spreadsheet and manually mark the ones you want to consider as bookmarks, so you can then search only those.
For even more functionality, you can send the results to a generative AI chatbot and ask natural language questions instead of searching for specific words.
Let’s get started!
Step 1. Install the atrrr R package
I suggest installing the development version of the atrrr R package from GitHub. I typically use the remotes package for installing from GitHub, although there are other options such as devtools or pak.
remotes::install_github("JBGruber/atrrr", build_vignettes = TRUE)
I’ll also be using dplyr, purrr, stringr, tidyr, and rio for data wrangling and DT to optionally display the data in a searchable table. Install those from CRAN, then load them all with the following code.
library(atrrr)
library(dplyr)
library(purrr)
library(stringr)
library(tidyr)
library(rio)
library(DT) # optional
You will need a free Bluesky application password (different from your account password) to request data from the Bluesky API. The first time you make a request via atrrr, the app should helpfully open the correct page on the Bluesky website for you to add an app password, if you’re already logged into Bluesky in your default browser. Or, you can go here to create an app password in advance.
Either way, when you first use the atrrr package, you’ll be asked to input an application password if there’s not one already stored. Once you do that, a token should be generated and stored in a cache directory at file.path(tools::R_user_dir("atrrr", "cache"), Sys.getenv("BSKY_TOKEN", unset = "token.rds"))
. Future requests should access that token automatically.
You can find more details about Bluesky authentication at the atrrr package website.
Step 2. Download and wrangle your Bluesky likes
The get_actor_likes()
function lets you download a user’s likes. Here’s how to download your 100 most recent likes:
my_recent_likes
Note: As of this writing, if you ask for more likes than there actually are, you’ll get an error. If that happens, try a smaller number. (You may end up with a larger number anyway.)
The get_actor_likes()
command above returns a data frame — more specifically a tibble, which is a special type of tidyverse data frame — with 20 columns. However, some of those columns are list columns with nested data, which you can see if you run glimpse(my_recent_likes)
. You’ll probably want to extract some information from those.
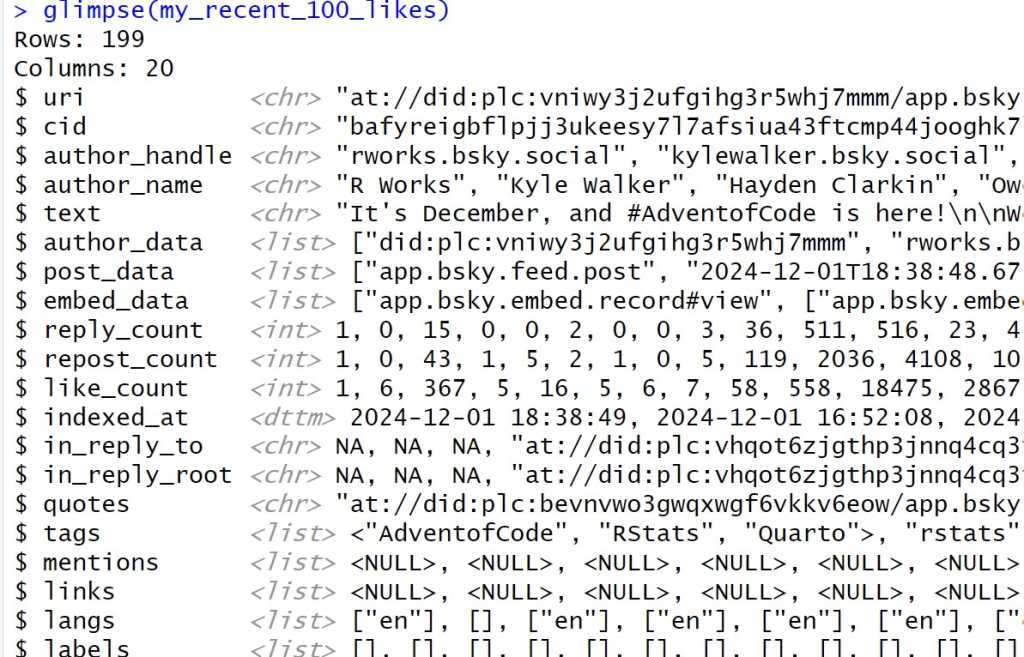
IDG
As shown above, each liked post’s text, author_name, author_handle, like_count, and repost_count are arranged in conventional (unnested) columns. However, the timestamp when the post was created is nested inside the posted_data list column, while any URLs mentioned in a post are buried deep within the embed_data column.
Here’s one way to unnest those columns using the tidyr package’s unnest_wider()
function:
my_recent_likes_cleaned
unnest_wider(post_data, names_sep = "_", names_repair = "unique") |>
unnest_wider(embed_data, names_sep = "_", names_repair = "unique") |>
unnest_wider(embed_data_external, names_sep = "_", names_repair = "unique")
That creates a data frame (tibble) with 40 columns. I’ll select and rename the columns I want, and add a TimePulled timestamp column, which could come in handy later.
my_recent_likes_cleaned
select(Post = text, By = author_handle, Name = author_name, CreatedAt = post_data_createdAt, Likes = like_count, Reposts = repost_count, URI = uri, ExternalURL = embed_data_external_uri) |>
mutate(TimePulled = Sys.time() )
Another column that would be useful to have is the URL of each liked post. Note that the existing URI column is in a format like at://did:plc:oky5czdrnfjpqslsw2a5iclo/app.bsky.feed.post/3lbd2ee2qvm2r. But the post URL uses the syntax https://bsky.app/profile/{author_handle}/post/{a portion of the URI}.
The R code below creates a URL column from the URI and By columns, adding a new PostID column as well for the part of the URI used for the URL.
my_recent_likes_cleaned
mutate(PostID = stringr::str_replace(URI, "at.*?post\\/(.*?)$", "\\1"),
URL = glue::glue("https://bsky.app/profile/{By}/post/{PostID}") )
I typically save data like this as Parquet files with the rio package.
rio::export(my_recent_likes_cleaned, "my_likes.parquet")
You can save the data in a different format, such as an .rds file or a .csv file, simply by changing the file extension in the file name above.
Step 3. Keep your Bluesky likes file updated
It’s nice to have a collection of likes as a snapshot in time, but it’s more useful if you can keep it updated. There are probably more elegant ways to accomplish this, but a simple approach is to load the old data, pull some new data, find which rows aren’t in the old data frame, and add them to your old data.
previous_my_likes
Next, get your recent likes by running all the code we did above, up until saving the likes. Change the limit to what’s appropriate for your level of liking activity.
my_recent_likes
unnest_wider(post_data, names_sep = "_", names_repair = "unique") |>
unnest_wider(embed_data, names_sep = "_", names_repair = "unique") |>
unnest_wider(embed_data_external, names_sep = "_", names_repair = "unique") |>
select(Post = text, By = author_handle, Name = author_name, CreatedAt = post_data_createdAt, Likes = like_count, Reposts = repost_count, URI = uri, ExternalURL = embed_data_external_uri) |>
mutate(TimePulled = Sys.time() ) |>
mutate(PostID = stringr::str_replace(URI, "at.*?post\\/(.*?)$", "\\1"),
URL = glue::glue("https://bsky.app/profile/{By}/post/{PostID}") )
Find the new likes that aren’t already in the existing data:
new_my_likes
Combine the new and old data:
deduped_my_likes
And, finally, save the updated data by overwriting the old file:
rio::export(deduped_my_likes, 'my_likes.parquet')
Step 4. View and search your data the conventional way
I like to create a version of this data specifically to use in a searchable table. It includes a link at the end of each post’s text to the original post on Bluesky, letting me easily view any images, replies, parents, or threads that aren’t in a post’s plain text. I also remove some columns I don’t need in the table.
my_likes_for_table
mutate(
Post = str_glue("{Post} >>"),
ExternalURL = ifelse(!is.na(ExternalURL), str_glue("{substr(ExternalURL, 1, 25)}..."), "")
) |>
select(Post, Name, CreatedAt, ExternalURL)
Here’s one way to create a searchable HTML table of that data, using the DT package:
DT::datatable(my_likes_for_table, rownames = FALSE, filter = 'top', escape = FALSE, options = list(pageLength = 25, autoWidth = TRUE, filter = "top", lengthMenu = c(25, 50, 75, 100), searchHighlight = TRUE,
search = list(regex = TRUE)
)
)
This table has a table-wide search box at the top right and search filters for each column, so I can search for two terms in my table, such as the #rstats hashtag in the main search bar and then any post where the text contains LLM (the table’s search isn’t case sensitive) in the Post column filter bar. Or, because I enabled regular expression searching with the search = list(regex = TRUE)
option, I could use a single regexp lookahead pattern (?=.rstats)(?=.(LLM)
) in the search box.
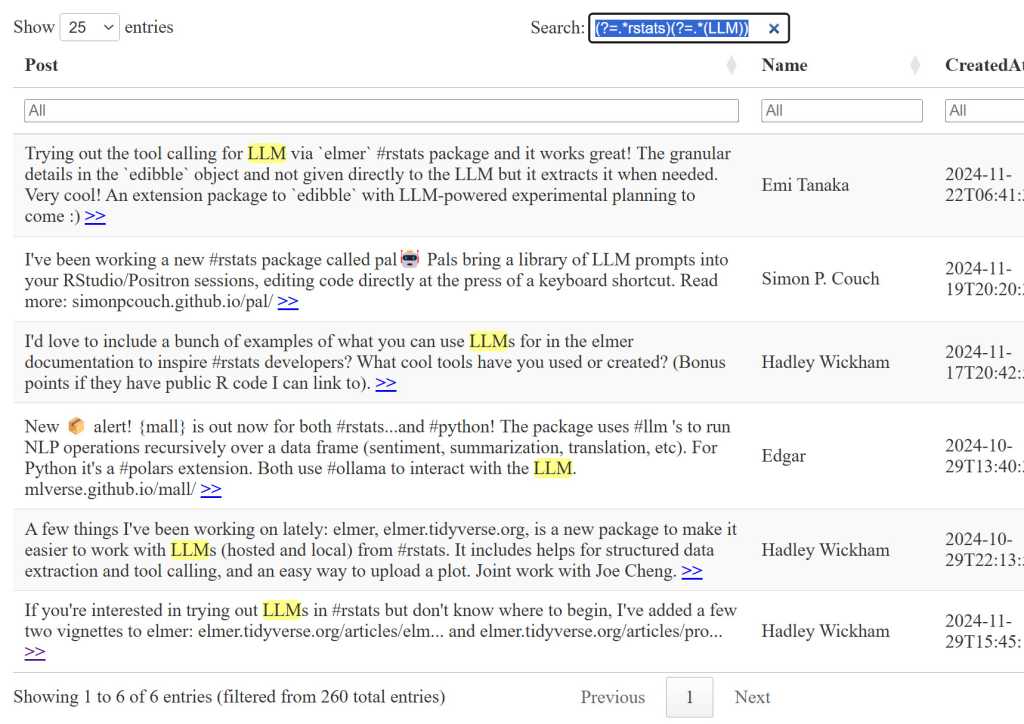
IDG
Generative AI chatbots like ChatGPT and Claude can be quite good at writing complex regular expressions. And with matching text highlights turned on in the table, it will be easy for you to see whether the regexp is doing what you want.
Query your Bluesky likes with an LLM
The simplest free way to use generative AI to query these posts is by uploading the data file to a service of your choice. I’ve had good results with Google’s NotebookLM, which is free and shows you the source text for its answers. NotebookLM has a generous file limit of 500,000 words or 200MB per source, and Google says it won’t train its large language models (LLMs) on your data.
The query “Someone talked about an R package with science-related color palettes” pulled up the exact post I was thinking of — one which I had liked and then re-posted with my own comments. And I didn’t have to give NotebookLLM my own prompts or instructions to tell it that I wanted to 1) use only that document for answers, and 2) see the source text it used to generate its response. All I had to do was ask my question.
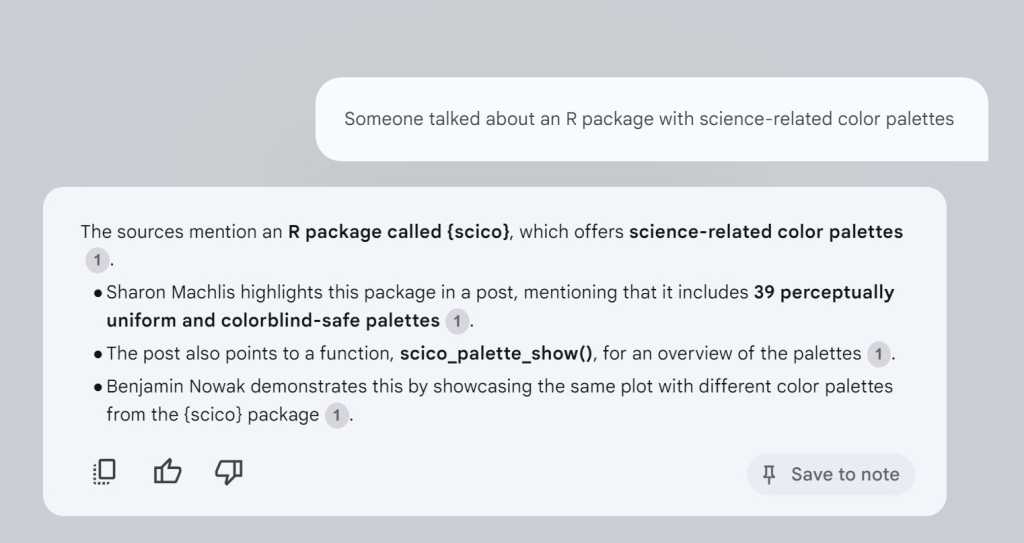
IDG
I formatted the data to be a bit more useful and less wasteful by limiting CreatedAt to dates without times, keeping the post URL as a separate column (instead of a clickable link with added HTML), and deleting the external URLs column. I saved that slimmer version as a .txt and not .csv file, since NotebookLM doesn’t handle .csv extentions.
my_likes_for_ai
mutate(CreatedAt = substr(CreatedAt, 1, 10)) |>
select(Post, Name, CreatedAt, URL)
rio::export(my_likes_for_ai, "my_likes_for_ai.txt")
After uploading your likes file to NotebookLM, you can ask questions right away once the file is processed.

IDG
If you really wanted to query the document within R instead of using an external service, one option is the Elmer Assistant, a project on GitHub. It should be fairly straightforward to modify its prompt and source info for your needs. However, I haven’t had great luck running this locally, even though I have a fairly robust Windows PC.
Update your likes by scheduling the script to run automatically
In order to be useful, you’ll need to keep the underlying “posts I’ve liked” data up to date. I run my script manually on my local machine periodically when I’m active on Bluesky, but you can also schedule the script to run automatically every day or once a week. Here are three options:
- Run a script locally. If you’re not too worried about your script always running on an exact schedule, tools such as taskscheduleR for Windows or cronR for Mac or Linux can help you run your R scripts automatically.
- Use GitHub Actions. Johannes Gruber, the author of the atrrr package, describes how he uses free GitHub Actions to run his R Bloggers Bluesky bot. His instructions can be modified for other R scripts.
- Run a script on a cloud server. Or you could use an instance on a public cloud such as Digital Ocean plus a cron job.
Use a spreadsheet or R tool to add Bookmarks and Notes columns
You may want a version of your Bluesky likes data that doesn’t include every post you’ve liked. Sometimes you may click like just to acknowledge you saw a post, or to encourage the author that people are reading, or because you found the post amusing but otherwise don’t expect you’ll want to find it again.
However, a caution: It can get onerous to manually mark bookmarks in a spreadsheet if you like a lot of posts, and you need to be committed to keep it up to date. There’s nothing wrong with searching through your entire database of likes instead of curating a subset with “bookmarks.”
That said, here’s a version of the process I’ve been using. For the initial setup, I suggest using an Excel or .csv file.
Step 1. Import your likes into a spreadsheet and add columns
I’ll start by importing the my_likes.parquet file and adding empty Bookmark and Notes columns, and then saving that to a new file.
my_likes
mutate(Notes = as.character(""), .before = 1) |>
mutate(Bookmark = as.character(""), .after = Bookmark)
rio::export(likes_w_bookmarks, "likes_w_bookmarks.xlsx")
After some experimenting, I opted to have a Bookmark column as characters, where I can add just “T” or “F” in a spreadsheet, and not a logical TRUE or FALSE column. With characters, I don’t have to worry whether R’s Boolean fields will translate properly if I decide to use this data outside of R. The Notes column lets me add text to explain why I might want to find something again.
Next is the manual part of the process: marking which likes you want to keep as bookmarks. Opening this in a spreadsheet is convenient because you can click and drag F or T down multiple cells at a time. If you have a lot of likes already, this may be tedious! You could decide to mark them all “F” for now and start bookmarking manually going forward, which may be less onerous.
Save the file manually back to likes_w_bookmarks.xlsx.
Step 2. Keep your spreadsheet in sync with your likes
After that initial setup, you’ll want to keep the spreadsheet in sync with the data as it gets updated. Here’s one way to implement that.
After updating the new deduped_my_likes likes file, create a bookmark check lookup, and then join that with your deduped likes file.
bookmark_check
select(URL, Bookmark, Notes)
my_likes_w_bookmarks
relocate(Bookmark, Notes)
Now you have a file with the new likes data joined with your existing bookmarks data, with entries at the top having no Bookmark or Notes entries yet. Save that to your spreadsheet file.
rio::export(my_likes_w_bookmarks, "likes_w_bookmarks.xlsx")
An alternative to this somewhat manual and intensive process could be using dplyr::filter()
on your deduped likes data frame to remove items you know you won’t want again, such as posts mentioning a favorite sports team or posts on certain dates when you know you focused on a topic you don’t need to revisit.
Next steps
Want to search your own posts as well? You can pull them via the Bluesky API in a similar workflow using atrrr’s get_skeets_authored_by()
function. Once you start down this road, you’ll see there’s a lot more you can do. And you’ll likely have company among R users.
Getting started with Azure Managed Redis 19 Dec 2024, 10:00 am
Microsoft has made a lot of big bets in its preferred cloud-native infrastructure. You only need to look at .NET Aspire and Radius to see how the company thinks you should be designing and building code: a growing cloud-native stack that builds on Kubernetes and associated tools to quickly build distributed applications at scale.
Azure CTO Mark Russinovich has regularly talked about one overarching goal for Azure: to make everything serverless. It’s why many tools we used to install on dedicated servers are now offered as managed services and Azure manages the underlying infrastructure. Services like Azure Container Instances allow you to quickly deploy Kubernetes applications, but what of the other services necessary to build at-scale containerized applications?
One key service is a caching in-memory database. Here Azure has relied on Redis, providing support for the Redis enterprise release as well as offering Azure Cache for Redis based on the lower-performance Redis community edition.
If you’re building a large application, you need more than a single-threaded database, as you’re leaving compute capabilities on the table. Azure Cache for Redis is unlikely to use all of the capabilities of the underlying vCPUs. As it requires two vCPUs for each instance, one primary and one replica for backup or failover, Azure Cache for Redis is not economical to use at scale.
Introducing Azure Managed Redis
At Ignite 2024, Microsoft announced the public preview of a managed service based on Redis Enterprise, taking advantage of it to improve performance so you can deliver bigger applications without requiring significant additional infrastructure. With data center power usage increasingly an issue, improving performance without increasing the number of required vCPUs helps Azure use its underlying servers more efficiently.
Shipping in preview as Azure Managed Redis, this new service is a higher-performance alternative to Azure Cache for Redis. It takes advantage of a new architecture that should significantly improve operations and support new deployment options.
Instead of one instance per VM, you’re now able to stack multiple instances behind a Redis proxy. There’s another big change: Although you still use two nodes, both nodes run a mix of primary and replica processes. A primary instance uses more resources than a replica, so this approach lets you get the best possible performance out of your VMs. At the same time, this mix of primary and replica nodes automatically clusters data to speed up access and enable support for geo-replication across regions.
Azure Managed Redis has two different clustering policies, OSS and Enterprise. The OSS option is the same as used by the community edition, with direct connections to individual shards. This works well, with close-to-linear scaling, but it does require specific support in any client libraries you’re using in your code. The alternative, Enterprise, works through a single proxy node, simplifying connection requirements for clients at the expense of performance.
Why would you use Redis in an application? In many cases it’s a tool for keeping regularly accessed data cached in memory, allowing quick read/write access. It’s being used any place you need a fast key/value store with support for modern features such as vector indexing. Using Redis as an in-memory vector index helps keep latency to a minimum in AI applications based on retrieval-augmented generation (RAG). Cloud-native applications can use Redis as a session store to manage state across container applications, so AI applications can use Redis as a cache for recent output, using it as semantic memory in frameworks like Semantic Kernel.
Setting up Azure Managed Redis
Microsoft is offering four different tiers that support different use cases and balance cost and performance. The lowest-cost option, best suited for development and test, is the memory-optimized tier. Here you have an 8:1 memory to vCPU ratio that works best when you need to store a lot of data for quick access but don’t need to process it significantly. The downside is a lower throughput, something that’s likely to be more of an issue in production.
Most applications will use the balanced option. This has a 4:1 memory to vCPU ratio, which will allow you to work with cached data as well as deliver it to applications. If you need more performance, there’s a compute-optimized tier, with a 2:1 memory to vCPU ratio, making it ideal for high-performance applications that may not need to cache a lot of data.
There’s one final configuration intended for applications that need to cache a lot of data, using NVMe flash to store data that’s not needed as often. These instances automatically tier your data for you, and although there may be a performance hit, it keeps the costs of large instances to a minimum. The architecture of this tier is interesting: It keeps all the keys in RAM and only stores values in flash when they’re found to be “cold.”
This approach is different from the standard data persistence feature, which makes a disk backup of in-memory data that can recover an instance if there’s an outage. Using this feature writes data to disk and only reads it when necessary; normal operations continue to be purely in-memory.
Once you have chosen the type of service you’re implementing, you can add it to an Azure resource group, using the Azure Portal. Start by creating a new resource and choosing the Redis Cache option from Databases. It’s important to note that there’s no explicit Azure Managed Redis control plane; it shares the same basic configuration screen as the existing Azure Cache for Redis. You’re not limited to using the portal, as there are Azure CLI, Bicep, and PowerShell options.
On the New Redis Cache screen, you’ll need to choose a Managed Redis SKU to unlock its configuration tools. This isn’t particularly clear, and you can easily miss this choice and accidentally pick the default Azure Cache for Redis settings. Once you’ve chosen a correct SKU, you can add modules from the Advanced tab, along with configuring the clustering policy and whether you’re using data persistence. You can now click Create and your instance will spin up. This can take some time, but once it’s running, it’s ready for use.
Choosing memory and compute
Within each tier are different memory and vCPU options, allowing you to choose the configuration that’s best for your application. For example, a balanced system starts with 500MB of storage and 2 vCPUs, with up to 1TB of storage and 256 vCPUs. Each option has its own list of storage and compute options, allowing you to dial in a specification that fits your workloads.
Available modules include support for search, time-series content, as well as JSON. It’s important to check that they work with your choice of SKU: If you’re using flash-optimized instances, you can’t use the time-series module or the Bloom probabilistic data structures, which are designed for working with streaming data.
Once configured you won’t be able to change many of its policies. Clustering, geo-replication, and modules are all configured during setup, and any changes require completely rebuilding your Redis setup from scratch. That’s not an issue for development and test, but it’s something to consider when running in production.
If you’re already using Azure Cache for Redis in your applications, you won’t need to make many changes to your code. The same Redis clients work for the new service, so all you need to do is configure the appropriate endpoints and use familiar query APIs. Azure recommends the StackExchange.Redis library for .NET developers. Redis keeps a list of its own client libraries for other languages, from Python to Java and more.
Azure’s latest Redis offering brings enterprise capabilities beyond its existing Azure Cache for Redis. This should help you build larger, more flexible cloud-native applications, as well as use in-memory data for more than simple key/value caching. As Azure Managed Redis moves towards general availability, hopefully we‘ll see Microsoft‘s cloud-native development frameworks like Aspire and Radius add support for automatically configuring its new services alongside their existing Redis support.
FAQ: Getting started with Bluesky 19 Dec 2024, 10:00 am
What is Bluesky?
Bluesky is a “microblogging” social media network similar to X (formerly Twitter), where people can publish posts with a maximum of 300 characters, as well as follow others, create lists, like posts, and so on. “Similar to Twitter” makes sense, because Bluesky was originally a project within Twitter to develop a more open and decentralized social network. Bluesky’s AT Protocol is open source.
Bluesky started as an invitation-only network last year and opened to the public in early 2024.
Why Bluesky?
The Bluesky network’s recent mainstream prominence is due largely to users leaving X over decisions by owner Elon Musk. But there’s a lot more to Bluesky than US politics.
Bluesky has a mix of technical and general discussions, with accounts like the Linux Foundation, Hugging Face, and xkcd comic creator Randall Munro, along with more mainstream organizations such as CNN, the Financial Times, and The Onion. I’ve even found some local TV meteorologists.
The platform’s appeal includes user-created “starter packs” that make it easy to find and follow people by topic, robust moderation tools, and the option to view posts either chronologically or via built-in or user-generated feeds.
These features have encouraged creation of communities-within-communities like Blacksky, launched by technologist Rudy Fraser. That project aims to offer content “created by and for Black users” as well as moderation tools designed to preemptively block online harassment that many Black users often experience.
Two of the chief complaints against X are the difficulty many users had in seeing content they actually wanted and the inability to control online harassment. Stopping harassment and hate speech remains imperfect at Bluesky, too, but Bluesky gives users more control over both — without making them do all the blocking and reporting themselves. Instead, users can subscribe to block lists and labeling tools created by others whom they trust.
“Shoutout to @blacksky.app,” one user posted recently. “A racist account just tried to interact with me, the content was blocked by Blacksky. I didn’t even have to see it. I feel safe for the first time in a long time online.”
How do I get started as a user?
One of Bluesky’s advantages compared to Mastodon, another decentralized open-source option, is its easy onboarding. Simply head to https://bsky.app and sign up. (Mastodon users need to choose a server, which can be a deal-breaker for those who don’t want to research server advantages before joining a network.)
You can choose a username at the default bsky.social domain, such as my @smachlis.bsky.social. Or, you can use a web domain you control as your Bluesky user name, such as @linuxfoundation.org. See “How to verify your Bluesky account” for instructions.
How can I find people to follow?
Besides the slow, manual way of searching for accounts you follow elsewhere or scrolling the Discover feed to see accounts of interest, some tools can help.
User-created started packs, mentioned above, will let you find people based on various topics. You can use Bluesky’s search to look for phrases such as Rstats starter pack or Python starter pack. Or, there are third-party searchable starter pack listings such as Bluesky Directory’s. You can subscribe to all users in a starter pack with one click or decide whether to follow each account individually.
If you were previously on X, there’s a Chrome extension called Sky Follower Bridge that lets you search for people you followed there to follow on Bluesky.
Feeds are another way to find content by topic. Third-party tools such as Bluesky Feeds have been a bit fragile recently as usage soars, but they may still be worth a try. Another way to find feeds is to look at the Feeds tab on a user’s profile page to see if they have any of interest. For example, I built a Fave data hashtags feed that looks for #RStats, #QuartoPub, #RLadies, or #PyLadies as well as the non-hashtag DuckDB. It uses the free Skyfeed Builder (and can be slow to load).
As with most social networks, you can also view posts with a specific hashtag. I just bookmark these in my browser, such as https://bsky.app/hashtag/rstats for rstats (case doesn’t matter).
There are a lot more Bluesky tools listed at the GitHub repo Awesome Bluesky.
But don’t discount the Discover feed, which is somewhat customizable. As you scroll that feed, you can click the three dots on a post to see an additional menu that includes options to “Show more like this” or “Show less like this.”
How do I subscribe to moderation and labeling lists?
Once you find a labeler, it’s a simple matter of clicking the subscribe button. Once you do, you can choose to hide, be warned, or disable each label’s posts. Moderation lists let you report a post to the list’s moderation, not relying solely on Bluesky’s main team.
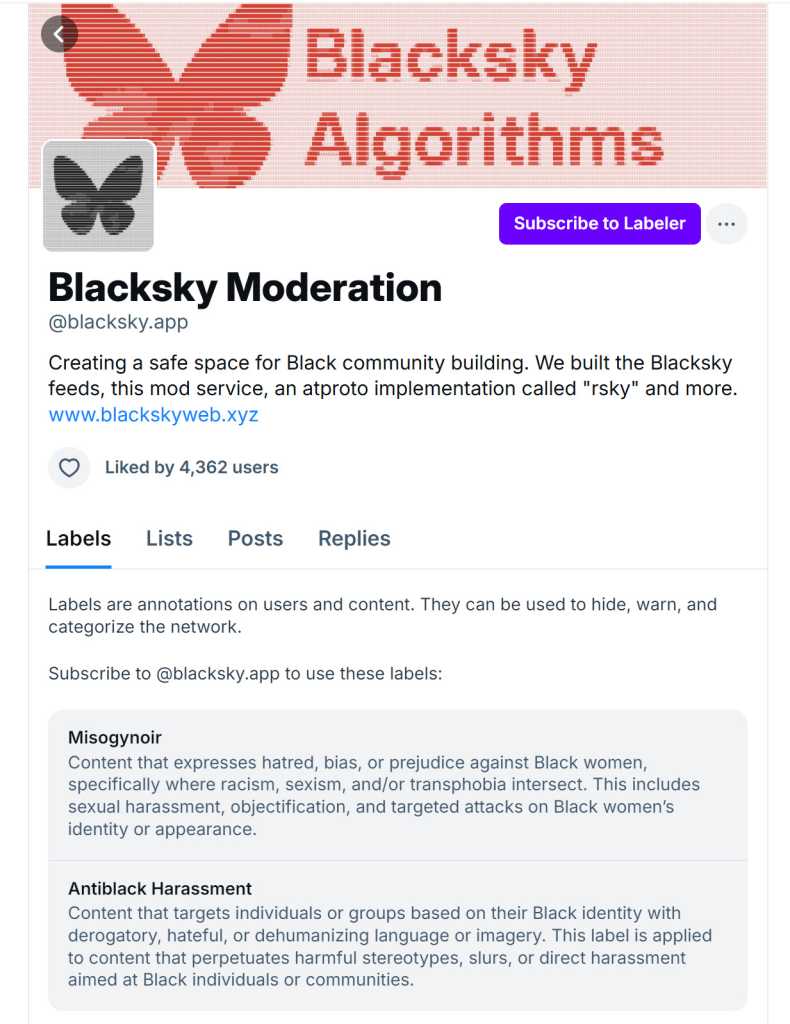
IDG
The third-party BlueSky Labelers site has a directory of some labelers. Some of these are used for moderation and others for matters of interest, such as verifying Microsoft employees or labeling GitHub repositories you contribute to.
Who owns Bluesky?
Bluesky spun off from Twitter in 2021 and is currently a Public Benefit Corporation (similar to Posit, formerly RStudio), which means it can legally take the interests of its user community into account and not only its owners. The Bluesky site says the company is owned by “[CEO] Jay Graber and the Bluesky team,” although it has also announced investments from companies including Blockchain Capital (it says it is not getting involved in crypto).
Bluesky executives have said that since its underlying protocol is open source, if something were to happen to its ownership, another entity could spin up infrastructure and allow people to port their accounts to another server. However, Mastodon advocates argue that the same single server and ownership team that make Bluesky easier to use, also increase the risk of the service being sold as Twitter was.
How does Bluesky make money?
Bluesky doesn’t run ads. The company says it has raised $15 million in Series A financing and has plans for a premium user subscription service. Paying subscribers won’t get any kind of algorithm boost, but they could get additional features such as posting higher-quality videos or customized profiles.
How can I build something around Bluesky?
For those of us who like to code, there’s a free API. “Bluesky is an open ecosystem with an API that is guaranteed to always be open to developers,” CEO Jay Graber posted. Bluesky’s underlying protocol is open source under the MIT or Apache Version 2.0 license. (Twitter started charging for its API in February 2023.)
You can code your own custom feeds to use and share or use third-party tools like SkyFeed, which author @redsolver.dev says will be open-sourced “soon.”
Bluesky has starter templates for building bots, custom feeds, and client apps.
For R users, there are two packages, atrrr and bskyr, not currently listed on the official SDK page. See my companion article on using atrrr to create a searchable collection of Bluesky bookmarks. Check the Bluesky documentation to see options in TypeScript, Python, Dart, and Go.
How big is Bluesky?
While still tiny in total users compared to alternatives like X or Threads, Bluesky is growing. The platform jumped from around 12 million registered users in mid-October to more than 24 million today. Total visits to the bsky.app website, which excludes mobile apps and other third-party tools, soared to 122.7 million in November vs. 75.8 million in October.
Some in the media say they’re getting more engagement on the platform than sites that are larger. “Traffic from Bluesky to @bostonglobe.com is already 3x that of Threads, and we are seeing 4.5x the conversions to paying digital subscribers,” posted Matt Karolian, vice president of platforms and R&D at the Boston Globe.
GitHub launches free tier of Copilot AI coding assistant 18 Dec 2024, 11:19 pm
GitHub has launched GitHub Copilot Free, providing access to a free tier of the AI-based pair programmer via Microsoft’s Visual Studio Code editor.
Announced December 18, Copilot Free gives developers access to 2,000 code completions and 50 chat messages monthly. The service requires signing in with a personal GitHub account or creating a new one. Developers may choose between Anthropic’s Claude 3.5 Sonnet or OpenAI’s GPT-4o models. It’s possible to ask coding questions or find bugs, including searches across multiple files. Developers can also access Copilot third-party agents or build their own extensions.
Copilot Free is automatically integrated into VS Code. To access the free tier, VS Code users can press the Copilot icon at the top of the screen and click the “Sign in to use Copilot for Free” button. This opens a web browser where developers can sign in to GitHub and allow VS Code to connect to their account. VS Code will then automatically install GitHub Copilot. GitHub is launching Copilot Free in response to having passed 150 million developers on the GitHub platform.
OpenAI rolls out upgrade to reasoning model, new dev tools 18 Dec 2024, 10:34 pm
The release Tuesday by OpenAI of OpenA1 o1, its reasoning model, in API, along with three new sets of tools for developers, is a “smart move that keeps [it] in the corporate/enterprise conversation, as so many customers are shifting their focus towards agentic applications over simpler chat-based applications,” an industry analyst said.
Jason Andersen, VP and principal analyst with Moor Insights & Strategies, also described it as “something of a defensive move, since we have seen AWS, Google, and Microsoft releasing API-based multi-model development frameworks such as Bedrock and AI Foundry.”
Some of those frameworks, he said, “do have OpenAI as a potential model target, but they also have new features such as model routing, which means that there is no guarantee that the framework vendor will consistently use OpenAPI.”
Released as part of its 12 days of Shipmas campaign, OpenAI o1 in API is production ready, the company said, and contains vision capabilities that allow it to “reason over images to unlock many more applications in science, manufacturing, or coding, where visual inputs matter.” Other enhancements include function calling to connect o1 to external data and APIs, as well as an API parameter that gives a developer the ability to “control how long model thinks before answering.” It is available to Tier 5 in the API.
Other additions to the toolset include enhancements to the Realtime API, which, OpenAI said, “allows developers to create low-latency, multi-modal conversational experiences. It currently supports both text and audio as inputs and outputs, as well as function calling capabilities.”
Key among them is integration with WebRTC, an open standard that makes it easier to build and scale real-time voice products across platforms. “Our WebRTC integration is designed to enable smooth and responsive interactions in real-world conditions, even with variable network quality,” the company said.
Also launched were Preference Fine Tuning, which it described as a new model customization technique that makes it easier to tailor models based on user and developer preferences, and new SDKs for Go and Java, both available in beta.
Andersen described the latter as “quite interesting, since those are two languages heavily used by developers who are using the public cloud. This and the APIs provide a meaningful alternative to someone just using the models a cloud provider has ‘on the shelf.’”
However, Forrester senior analyst Andrew Cornwall said, “when OpenAI says, ‘new tools for developers,’ they mean tools for AI developers — everyone else will start seeing things only after the AI developers get their hands on the goodies. That will take some time, since o1-2024-12-17 APIs are rolling out only to Tier 5 (their highest-usage users) today.”
He said that, looking at what was announced, “probably of most interest is vision support, a capability not in o1-preview. In addition, the latest o1 model should return more accurate responses and report fewer refusals than the preview.”
Math and coding capabilities, said Cornwall, “have improved from the preview, in some cases significantly — although o1’s reported score won’t dethrone Amazon Q as leader of the SWE-bench Verified leaderboard. OpenAI has also improved function calling and structured output — the abilities to call into a program from an AI query, and to return output in developer-friendly JSON rather than natural language.”
In addition, “[developers have] a new parameter to play with, reasoning effort, which will let them adjust between accuracy and speed,” he noted. “This will integrate nicely with WebRTC voice support — a pause for a few seconds is sometimes acceptable at a keyboard, but not when you’re having a conversation.”
He added, “more developers will get a chance to play with OpenAI’s most powerful model, with SDKs for Java and Go moving from alpha to beta. Businesses are eager to use AI, but many have been waiting for the stability that a beta might afford.”
“In short,” Cornwall said, “this isn’t revolutionary — most of the capabilities have been available in different models already — but with this release, a single model gets better at many modes and for many classes of problem.”
Added Thomas Randall, director of AI market research at Info-Tech Research Group, “with increased openness and flexibility for developers, OpenAI is aiming to maintain its market share as the go-to model provider for builders. Deeper dependencies may form between OpenAI’s model provision and the software companies that are already utilizing OpenAI for their own AI-enhanced offering.”
Tabnine code assistant now flags unlicensed code 18 Dec 2024, 9:55 pm
Looking to minimize IP liability for generative AI output, Tabnine’s AI coding assistant now checks code for licensing restrictions.
The Code Provenance and Attribution capability added to the tool enables enterprise developers to use large language models (LLMs) while minimizing the possibility of restrictively licensed code being injected into a codebase. With this new feature, Tabnine will more easily support development teams and their legal and compliance teams who wish to leverage a variety of models, the company said.
Now in private preview, the Provenance and Attribution capability was announced on December 17. Tabnine now can check code generated using AI chat or AI agents against code publicly visible on GitHub. It then flags any matches and references the source repository and its license type. This detail makes it easier for engineering teams to review code being generated with the assistance of AI and decide if the license of that code meets specific requirements and standards, Tabnine said.
Models trained on larger pools of data outside of permissively licensed open source code can provide superior performance, but enterprises using them run the risk of running afoul of IP and copyright violations, Tabnine president Peter Guagenti said. The Code Provenance and Attribution capability addresses this tradeoff and increases productivity while not sacrificing compliance, according to Guagenti. And, with copyright law for using AI-generated content still unsettled, Tabnine’s proactive stance aims to reduce the risk of IP infringement when enterprises use models such as Anthropic’s Claude, OpenAI’s GPT-4o, and Cohere’s Command R+ for software development.
The Code Provenance and Attribution capability supports software development activities including code generation, code fixing, generating test cases, and implementing Jira issues. Future plans include allowing users to identify specific repositories, such as those maintained by competitors, for generated code checks. Tabnine also plans to add a censorship capability to allow administrators to remove matching code before it is displayed to the developer.
From surveillance to safety: How Kazakhstan’s Carpet CCTV is reshaping security 18 Dec 2024, 10:30 am
In a world where technology increasingly shapes how cities manage safety and security, Kazakhstan’s Ministry of Internal Affairs is leading the way with its groundbreaking “Carpet CCTV” project. This ambitious initiative has revolutionized public safety by combining a massive surveillance network with advanced analytics and artificial intelligence, creating a system that shifts the focus from reactive responses to proactive prevention.
Over the past four years, the scope of Kazakhstan’s surveillance infrastructure has expanded dramatically. The number of cameras has grown from just 40,500 to an impressive 1.3 million, with 313,000 cameras now directly accessible to police. These cameras are strategically positioned to monitor key areas, enhancing law enforcement’s ability to detect, prevent, and respond to incidents in real time. The system has already shown its effectiveness: since early 2024, it has detected over 8,200 criminal offenses and recorded 7.1 million traffic violations, resulting in significant improvements in public safety and road management.
At the heart of this transformation is the use of artificial intelligence. By integrating cutting-edge technologies such as facial recognition, license plate detection, and crowd monitoring, the system provides actionable insights that allow authorities to address risks before they escalate. For example, facial recognition capabilities enable real-time identification of persons of interest, while AI-powered traffic monitoring contributes to improved road safety and generates public revenue through fines. These features highlight the system’s ability to go beyond passive recording, transforming it into a dynamic tool for crime prevention and urban management.
The implementation of the Carpet CCTV project, however, was not without challenges. Managing the enormous volume of data generated by over a million high-definition cameras required significant upgrades in communication networks and data storage infrastructure. The integration of public and private camera networks demanded a unified approach to data sharing and management, while privacy concerns necessitated robust regulatory frameworks to ensure citizen trust. Through a combination of strategic planning, public-private partnerships, and transparent communication, the Ministry successfully addressed these obstacles, setting a model for other nations to follow.
One of the project’s most significant achievements lies in its deterrent effect. Administrative offenses, such as public disturbances, have decreased sharply, indicating that the visible presence of surveillance cameras is influencing behavior. This demonstrates the power of technology not just to react to incidents, but to prevent them altogether. Furthermore, the use of video evidence has increased case resolution rates, further solidifying the system’s impact on law enforcement effectiveness.
Looking ahead, Kazakhstan plans to build on the success of Carpet CCTV by expanding its geographic coverage and enhancing its analytical capabilities. New developments will focus on leveraging advanced AI to improve the accuracy and scope of surveillance, while also incorporating adaptive privacy measures to protect civil liberties. This forward-thinking approach ensures the system remains at the forefront of public safety technology, balancing innovation with accountability.
Kazakhstan’s Carpet CCTV project represents more than just an investment in technology—it’s a vision for smarter, safer cities. By blending state-of-the-art solutions with thoughtful governance, the Ministry of Internal Affairs has created a system that not only addresses today’s challenges but also lays the groundwork for a secure and sustainable future.
For those interested in learning more about this transformative initiative, the full spotlight paper offers an in-depth exploration of the strategies and technologies behind its success.
Build a server-side web app with .NET, C#, and HTMX 18 Dec 2024, 10:00 am
There are many stacks on the server side and one of the most longstanding and capable ones is the .NET platform using C#. .NET is broadly comparable to the Java platform, and over the years, the two have been competitors and mutual influencers. Each draws inspiration from the other. In this article, we’ll develop a simple server-side generated application using C#. We’ll throw in a dash of HTMX for dynamic front-end interactivity without the JavaScript.
Create a .NET C# project
To start, you’ll want the .NET CLI tool installed on your system, which is a fairly painless procedure. Once that’s done, you’ll have a dotnet
command, which you can use to start a new application like so:
$ dotnet new mvc -n QuoteApp
This command creates a /QuoteApp
directory. If we move into the new directory, we’ll see a simple project laid out for us. We can run it in dev mode like so:
/QuoteApp $ dotnet run
If you visit localhost:5265
, you’ll see the starter screen like the one below:
If you need to change the port or accept incoming requests from a domain other than localhost
, you can modify the QuoteApp/Properties/launchSettings.json
. As an example, in the http
section, I modified the application to accept all incoming requests at port 3000:
"applicationUrl": "http://*:3000"
The model class
The layout of a typical .NET C# MVC application is similar to a Java Spring MVC application. Based on the Model View Controller design pattern, the first thing we want to do is add a model class. To start, create a new /Model
directory (.NET conventionally capitalizes directory and file names) and then a QuoteItem.cs
file:
// Models/Quote.cs
namespace QuoteApp.Models
{
public class Quote
{
public int Id { get; set; }
public string Text { get; set; }
public string Author { get; set; }
}
}
This yields a simple data class to hold the Id, Text, and Author fields for our sample application. Java developers will notice the use of get;
and set;
shorthand to create the corresponding getter and setter for each field.
.NET’s namespace concept is similar to Java’s package, although packages in Java have lowercase names by convention. In our case, the directory structure and namespace agree, although this is not strictly required in C#.
The repository class
We’ll use a repository class for persisting the quotes users submit to our application. In a real application, the repository class would interact with a datastore. For our example, we’ll just use an in-memory list. Since our application is small, we can put the repository class directly into our root directory for now.
Here’s the repository class:
// QuoteRepository.cs
using QuoteApp.Models;
namespace QuoteApp
{
public class QuoteRepository
{
private static List _quotes = new List()
{
new Quote { Id = 1, Text = "There is no try. Do or do not.", Author = "Yoda" },
new Quote { Id = 2, Text = "Strive not to be a success, but rather to be of value.", Author = "Albert Einstein" }
};
public List GetAll()
{
return _quotes;
}
public void Add(Quote quote)
{
// Simple ID generation (in real app, use database ID generation)
quote.Id = _quotes.Any() ? _quotes.Max(q => q.Id) + 1 : 1;
_quotes.Add(quote);
}
}
}
We’ll use a static block to declare and populate a _quotes List
. Using that data, we provide two methods: GetAll()
and Add()
. GetAll()
simply returns the List
, while Add
inserts the new Quote
into it. We use a simple increment logic to create an ID for the new Quote
.
The controller class
Now we can move on to the controller, which provides the application’s service layer:
// Controllers/QuoteController.cs
using Microsoft.AspNetCore.Mvc;
using QuoteApp.Models;
namespace QuoteApp.Controllers
{
public class QuoteController : Controller
{
private QuoteRepository _repository = new QuoteRepository();
public IActionResult Index()
{
var quotes = _repository.GetAll();
return View(quotes);
}
[HttpPost]
public IActionResult Add(string text, string author)
{
if (!string.IsNullOrEmpty(text) && !string.IsNullOrEmpty(author))
{
var newQuote = new Quote { Text = text, Author = author };
_repository.Add(newQuote);
//return Partial("_QuoteItem", newQuote);
return PartialView("_QuoteItem", newQuote);
}
return BadRequest();
}
}
}
The controller extends the abstract .NET MVC Controller class. It imports a couple of namespaces, one is from the .NET MVC library, and the other is our Quote model from the previous section. We then create a repository instance as a private member and define two public methods on it: Index()
and Add()
.
Both methods return IActionResult. Index()
takes the default GET HTTP
method while Add()
uses the [HttpPost]
attribute to accept a POST
. Attributes are similar to Java’s annotations and can decorate classes. In C#’s case, they also decorate namespaces.
The Index()
and Add()
methods use functions from the MVC framework to form responses: View
, PartialView
, and BadRequest
. Notice that Add()
returns a partial view; this is because we are going to use HTMX to submit the new quote request with Ajax, and we’ll take the markup for just the new Quote and insert it on the front end. You’ll see this in the next section.
The Index()
method returns the view automatically associated with it, which is defined in the Views/Quote/Index.cshtml
file. The view, which we’ll look at next, is provided with the list of quotes from the repository.
The view class
Our humble example only has two views, the index page and the quote. Here’s the index:
// Views/Quote/Index.cshtml
@model List
Quotes
@foreach (var quote in Model)
{
@await Html.PartialAsync("_QuoteItem", quote)
}
The .cshtml
file is a view defined in the Razor format, which is a templating technology that lets you use code and data in an HTML file. This is similar to other templating solutions like JSP, Thymeleaf, or Pug. It’s plain HTML with a dash of HTMX except for the server-side bits where we do the following:
- Import the list of quotes provided by the controller, using
@model
- Use the list to iterate:
@foreach (var quote in Model)
- Render each quote in the list
Each quote is rendered with the line @await Html.PartialAsync("_QuoteItem", quote)
. PartialAsync means we are rendering a partial view (the quote template you’ll see shortly) using an asynchronous operation. Because it is async, we use the @await
directive to wait for the operation to finish.
The _QuoteItem
template is defined like so:
//Views/Quote/_QuoteItem.cshtml
@model QuoteApp.Models.Quote
@Model.Text - @Model.Author
This template takes the Quote
from the Add()
controller method as its model and uses it to render a simple list item.
The form
Back in Index.cshtml
, the form uses HTMX attributes to submit the fields in the background with Ajax. We’ll do the following:
- Send a
POST
Ajax request to.quote/add: hx-post="/quote/add"
- Put the response into the
#quoteList element: hx-target="#quoteList"
- Put the response before the end of the target:
hx-swap="beforeend"
The net result is that the markup sent back in the response from the Add()
service (the element) will be inserted directly at the end of the quote list
.
Launch the main program
Program.cs
is responsible for launching the program:
// QuoteApp/Program.cs
var builder = WebApplication.CreateBuilder(args);
// Add services to the container.
builder.Services.AddControllersWithViews();
var app = builder.Build();
// Configure the HTTP request pipeline.
if (!app.Environment.IsDevelopment())
{
app.UseExceptionHandler("/Home/Error");
// The default HSTS value is 30 days. You may want to change this for production scenarios, see https://aka.ms/aspnetcore-hsts.
app.UseHsts();
}
app.UseHttpsRedirection();
app.UseStaticFiles();
app.UseRouting();
app.UseAuthorization();
app.MapControllerRoute(
name: "default",
pattern: "{controller=Home}/{action=Index}/{id?}");
app.MapControllerRoute(
name: "quote",
pattern: "quote/{action=Index}/{id?}",
defaults: new { controller = "Quote" });
app.Run();
This main file starts a new MVC application with the command-line arguments: WebApplication.CreateBuilder(args)
. The app generator adds a strict transport security layer if the developmentMode
environment variable is not set. The site also redirects to https
and serves static files by default.
The UseRouting
call means we’ll partake of the MVC routing plugin, and the UseAuthorization
does the same for the MVC authorization plugin.
Finally, we add the default Controller
and our Quote
controller. Notice the controller = “Quote”
automatically maps to Controller/QuoteController
.
Conclusion
Our new application is simple, but it gets the job done. It will list the quotes already submitted and allow us to add new ones dynamically when run with $ dotnet run
, as shown here:
Overall, .Net with C# is a well-thought-out platform for building apps in the MVC style, and HTMX gives us low-overhead Ajax. Check out the csharp-htmx application repository to see all the code for the demo.
4 key concepts for Rust beginners 18 Dec 2024, 10:00 am
Few languages are as highly esteemed by developers as Rust, which promises robust memory safety without compromising speed. Rust also has a steep learning curve, though, and some of its programming concepts can feel like a barrier to knowing it well.
As an aid to the aspiring Rustaceans, here are four key concepts of the Rustosphere—the kinds of things a more experienced Rust developer might wish they knew from the beginning. Having a grasp on these concepts will give you a head start when you start writing programs with Rust.
Also see: Rust tutorial: Get started with the Rust language.
All Rust variables are immutable by default
Rust’s first assumption about any variable you create is that it is immutable by default.
If you say let x=1;
, the variable x
is bound to the value 1
for the lifetime of the program. Any variable that might change has to be specifically declared as such with the mut
(for “mutable”) keyword; e.g., let mut x=1;
.
This “Sir, may I?” strategy is a deliberate design choice for Rust, even though at first it might seem ornery. But here’s the rationale: Immutable entities in a program are easier to reason about and make guarantees about—especially guarantees about memory safety, which is Rust’s big selling point. If the compiler knows some element in a given scope doesn’t change once it’s assigned, most of the memory management issues around that element evaporate.
Another rationale for this design decision is that it forces the programmer to think about what actually does need to change in a program. Taking input from the user, for instance, or reading from a file, are operations that must be handled mutably. But you can leave many other operations as immutable with no loss of functionality.
With advanced Rust data structures, such as reference-counted Cell objects, you can use immutability to your advantage. For example, this immutable singly-linked list has no mutable components; every change to the list essentially involves creating a new list, albeit one that doesn’t waste memory copying because the existing list data can be re-used.
Rust has strict rules of ownership
Rust’s memory management model relies on a strict concept of ownership. Any value in Rust can only be modified by one thing at a time—its owner. Think of how there’s only one baseball at a time in a baseball game, and only one player at a time handling it. That owner can change over the lifetime of a program, in the same way the ball is thrown between players. But Rust refuses to allow a value to have more than one owner at once, just as we’d rather not have baseball players tussling over the ball.
Also see: Rust memory safety explained.
It’s possible for something to be read by more than one thing at once in Rust, but not possible for something to be changed by more than one thing at once. There can be multiple shared references or “borrows” to something at once, for the sake of read-only access, but there can be only one exclusive (read-write) reference to something at once.
The net effect is that Rust programmers must pay close attention to what data can be modified at what time. It’s important to understand that a program that breaks these rules won’t just crash; in Rust, it won’t even compile. That’s because the rules of ownership are not optional. (More on this later.)
Another net effect is that Rust memory management at runtime is not handled by way of a dedicated garbage collection system, as with C# or Go. Things like dynamic reference counting are possible, but they’re more akin to C++’s smart pointers—albeit with better runtime controls for thread safety.
A final note is that ownership does not make it impossible for Rust programs to have data race conditions. Data access across threads, for instance, still needs to be serialized with primitives like locks or queues to avoid unpredictable in-program behavior. But Rust does make it impossible for code to have many common memory-access-error bugs that plague other languages.
Borrow checking enforces Rust’s ownership rules
A big part of Rust’s appeal is that its ownership rules forbid representing states that might cause common memory errors. These problems aren’t caught at runtime but at compile time, so they never enter production.
A downside is that Rust programmers spend a fair amount of time, at least when they’re first learning the language, figuring out how to appease the compiler. Program behaviors that might have passed unnoticed in other languages (and possibly caused memory errors at runtime) cause the Rust compiler to stop cold.
The other big downside is that you can’t opt out of this behavior. You can’t toggle off Rust’s borrow-checking behavior the way you could, say, disable a code linter for another language. It makes for better software in the long run. But the immediate cost is a language that’s both slower to learn and slower to iterate in.
Rust does allow you to fence off parts of your code with the unsafe
keyword, and lift some restrictions, like the ability to dereference a raw pointer. But unsafe
does not turn off borrow checking entirely, or anything like that. It’s for taking code with certain behaviors you don’t want casually used (again, like dereferencing a raw pointer) and “gating” it for safety.
Also see: Safety off: Programming in Rust with ‘unsafe’.
Rust editions support backward-compatibility
It’s been almost a decade since Rust 1.0 came out, and the language has evolved aggressively since then. Many new features came along, not all of them backward-compatible with older versions of the language. To keep these changes from making it hard to maintain code in the long term, Rust introduced the concept of language editions.
Editions, which are published roughly every three years, provide a guarantee of compatibility. By and large, features added to Rust are supported going forward. Breaking changes—ones that are backward-incompatible—are added to the next edition rather than the current one.
Once you choose an edition, you’re not tied to it forever. You can migrate your code to future editions by way of both automated tooling and manual inspection. But there’s also a good chance the changes you are interested in will be backward-compatible with your older code, and thus available without migrating editions.
You have a license to code 18 Dec 2024, 10:00 am
One of the coolest things about software development is that you don’t need anyone’s permission to do it. If you need an application for your business or personal use, there isn’t a single thing stopping you from learning to code and writing it yourself. There’s no trade organization or governing body to seek approval from.
Now, if you want to practice law, or issue drug prescriptions, or even cut hair, you have to get permission from some government entity. But if you want to write the next viral application used by millions, you can just fire up your computer and start building. Nobody is going to stop you.
And more importantly, no one should stop you.
You don’t need a CS degree
Nor do you need to go to college to write code. For many professions, college and professional degrees are required. Sure, you could get a computer science degree, but I hold the somewhat contrary view that a CS degree isn’t nearly as valuable — or useful — as one might hope. It is an expensive way to learn something that you could teach yourself in a much shorter period of time. One can easily become a coding expert by watching YouTube videos, reading blogs, and looking at well-written code on GitHub.
In addition, a computer science degree normally doesn’t teach you many things you need to know to write code professionally. A CS student will learn about writing compilers and all kinds of grand theories about artificial intelligence, but probably not about the intricacies of Git and GitHub, or about how to write a good bug report. Standard algorithms and data structures are good to know, but the average developer doesn’t often write them. Few businesses will want you to spend time writing a doubly linked list when there is one ready to go in some standard library.
A much better solution these days would be a coding bootcamp. Cheaper, faster, and far more practical, bootcamps are the market response to what businesses need. They teach the actual skills that many (most?) software development shops are looking for today: JavaScript, TypeScript, React, HTML, CSS, Git, requirements gathering, and other “meta-programming” skills like bug reports and agile development.
This utter lack of control from higher authority comes from a simple fact: code is free and untameable. Not free like free beer, but free like the wind. You can’t stop someone from writing code. Any seven-year-old can build a game using Scratch or Python or Minecraft. Shoot, there are probably seven-year-olds writing better Python code than you or me. Your grandfather can break out Visual Studio Code and build a tool to track his stamp collection. I suppose that someone somewhere could try to regulate coding, but it seems like trying to bottle up chutzpah — it can’t be done.
This is true because no one can define the right way to build something in software, at least not in the same way we can define the right way to build a house or write a legally binding contract. I can’t imagine trying to impose a government-mandated set of rules for proper coding. It would be a disaster.
You don’t need a procedures manual
There is no “proper way” to code something, just like there is no proper way to paint a painting. Hand 100 teams the exact same design specification and you will get 100 wildly different implementations. Imagine telling Michelangelo, “No, I’m sorry, but that Sistine Chapel thing you did doesn’t conform to proper painting procedures and has to be redone under the supervision of our government inspector.” Yeah, right
I can’t imagine what trying to regulate software would do to innovation. Things move so fast in the software development world that no regulatory body could possibly keep up, and such an entity would almost certainly stifle any attempt to rapidly advance the industry. It seems obvious that the rapid rate of innovation comes as a result of the freedom software developers have to experiment with new ideas.
It’s never been a better time to be a smart, capable person. Eight hundred years ago, if you were ambitious, you had to get permission from a guild to do a long apprenticeship with a craftsman before you were allowed to strike out on your own.
Now? You can be up and running and learning and producing in a matter of hours. There’s nothing holding you back. While we all stand on the shoulders of giants, many of those giants started out creating things without any formal education. They just built the stuff that today enables us to be a coder if we want.
So go ahead — code like the wind.
JetBrains launches search portal for Kotlin Multiplatform libraries 17 Dec 2024, 11:50 pm
JetBrains has introduced klibs.io, a web service intended to make it easier to find libraries for Kotlin Multiplatform, a project that allows developers to build cross-platform mobile, desktop, and web apps from the same code base.
Now in an alpha state and billed as an experimental search platform for KMP (Kotlin Multiplatform) libraries, klibs.io was introduced December 17. The service enables developers to locate a Kotlin Multiplatform library for a specific purpose that supports selected platforms including the JVM, the Android JVM, WebAssembly, JavaScript, and Kotlin/Native. The website indexes data from Maven Central and GitHub, using AI-generated metadata to enhance search results.
A goal of klibs.io is making it easier to evaluate libraries by offering KMP-related information. Libraries range from the Coil image-loading library for Android to Arrow typed functional programming interfaces to the Kodein dependency retrieval container and even PeopleInSpace, a Kotlin Multiplatform sample project that lists people currently in space. A search engine attached to klibs.io cites more than 1,400 libraries available. Developers will spend less time searching while library authors benefit from increased visibility for their work, JetBrains said. Instructions for adding a library to kilbs.io are available in an FAQ.
Developers can participate in Slack to improve klibs.io. They also can sign up for Kotlin Slack via an application form. The klibs.io website was created by Kotlin developer advocate Ignat Beresnev and the Kotlin web team. Plans call for adding Java/JVM-only libraries at some point. Also eyed for kilbs.io is indexing of Gradle plugins as well as GitLab and Bitbucket support.
Page processed in 1.858 seconds.
Powered by SimplePie 1.3.1, Build 20121030095402. Run the SimplePie Compatibility Test. SimplePie is © 2004–2024, Ryan Parman and Geoffrey Sneddon, and licensed under the BSD License.